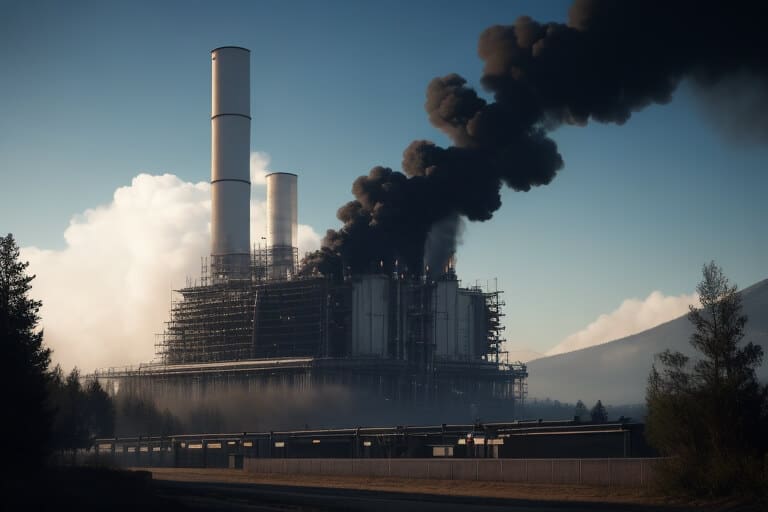
- December 7, 2023
- allix
- Research
You take steps to be eco-friendly: program your thermostat to cut down on heating bills, recycle your bottles and cans, cycle to work instead of drive, repeatedly use eco-friendly shopping bags, invest in solar energy, and even share showers with your partner—all to play your part in energy conservation, reducing rubbish, and shrinking your ecological mark.
New research could just throw a wrench in your efforts. A team from Carnegie Mellon University and Hugging Face, an online AI community, has found that even if you’re part of the over ten million individuals utilizing machine learning services every day, you might inadvertently contribute to environmental degradation.
In their recent research, considered the first in-depth analysis of environmental costs of machine-learning initiatives, the team discerned that producing one image through AI is as power-hungry as charging a smartphone battery.
“People often perceive AI as a zero-impact technological marvel floating in the ‘cloud’,” commented the research leader, Alexandra Luccioni. “Yet there’s an environmental price to pay each time we make an AI system perform tasks for us, and it’s critical to acknowledge this.”
Analysis of 30 datasets across 88 models revealed a vast discrepancy in energy usage dependent on the task. The researchers gauged the carbon emissions for each individual operation.
Topping the energy-consumption chart was Stability AI’s Stable Diffusion XL, a tool for creating pictures. Engaging this software generates close to 1,600 grams of carbon dioxide — about the same as driving a petrol vehicle for four miles. The energy expended for straightforward text creation was minimal, akin to driving a mere 3/500 of a mile.
The study spanned an assortment of machine learning tasks, including image and text classification, captioning pictures, summarizations, and answering queries.
The findings showed that generative activities which create new content—like visual graphics and text summaries—are more demanding in terms of energy and carbon output than classifying tasks, like movie ranking.
The use of versatile models for specific classification tasks was found to be less energy-efficient than dedicated models for the equivalent tasks — a vital point considering the current shift towards jack-of-all-trades models catered to a multitude of simultaneous tasks rather than specialized models fine-tuned for one specific operation.
“We’re especially struck by the implications of this finding, with the industry moving away from task-specific models towards versatile ones designed to handle various tasks in real-time,” stated the report.
Luccioni highlighted that for specific use-cases like parsing emails, such extensive all-purpose models might be unnecessary excess.
Although the carbon figures for these tasks may seem negligible, they can add up to a considerable environmental toll when you account for millions of AI users making repeated requests every day.
“In terms of AI that can generate new content, we ought to weigh its environmental costs against the benefits more carefully,” Luccioni advised.
Categories
- AI Education (37)
- AI in Business (62)
- AI Projects (85)
- Research (57)
Other posts
- The Complete List of 28 US AI Startups to Earn Over $100 Million in 2024
- Keras Model
- Scientists Develop AI Solution to Prevent Power Outages
- NBC Introduces AI-Powered Legendary Broadcaster for Olympic Games
- Runway Introduces Video AI Gen-3
- Horovod – Distributed Deep Learning with TensorFlow and PyTorch
- Research Reveals Contradictory Positions Of AI Models On Controversial Issues
- Using AI to Understand Dog Barking
- The Autobiographer App Uses Artificial Intelligence To Record Your Life Story
- Chainer – Dynamic Neural Networks For Efficient Deep Learning
Newsletter
Get regular updates on data science, artificial intelligence, machine