An Innovative Model Of Machine Learning Increases Reliability In Identifying Sources Of Fake News
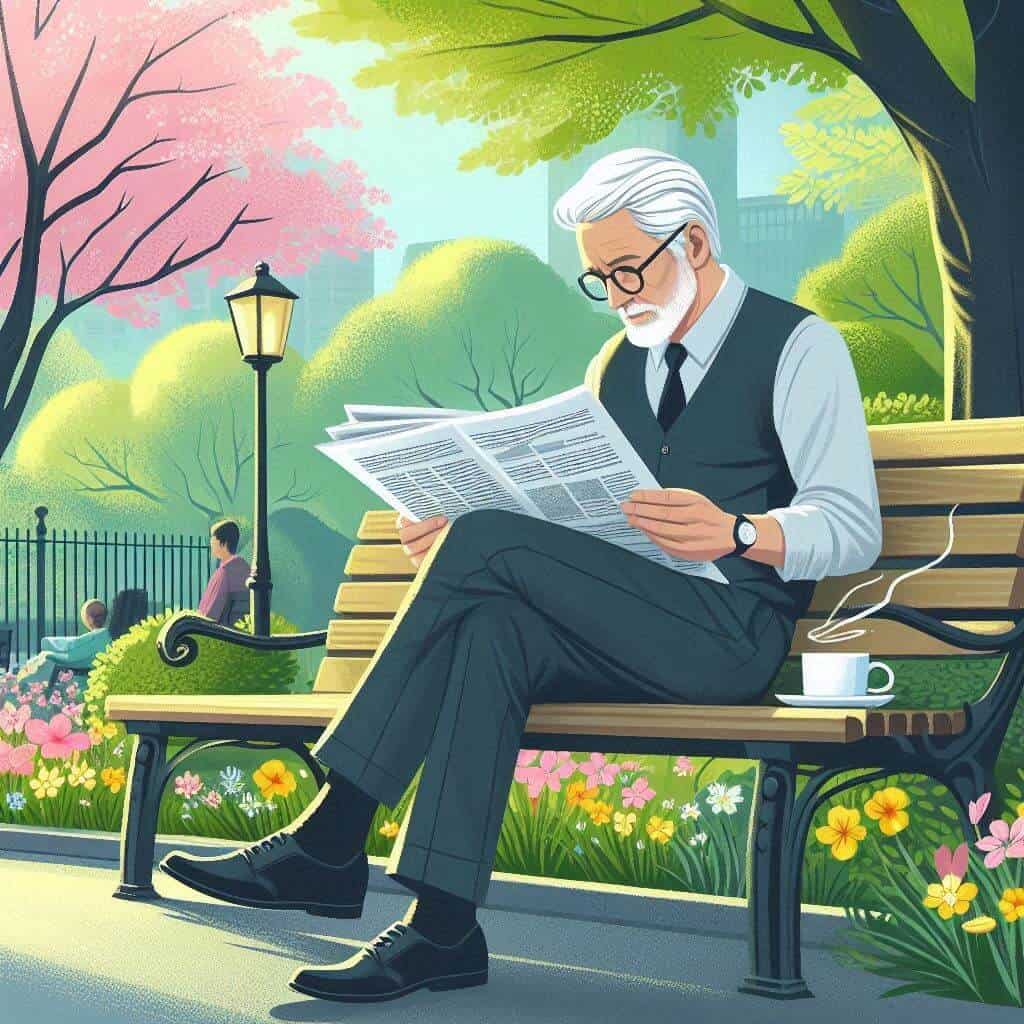
- October 31, 2024
- allix
- AI in Business
The problem of misinformation is an ongoing problem that is especially acute during elections, as conspiracies and misinformation spread by malicious actors attempt to sway voters. As the hotly contested U.S. election reaches its climax, researchers at Ben Gurion University of the Negev have unveiled a strategy to help fact-checkers deal with the growing tide of misinformation spreading on social media.
The team, led by Dr. Nir Greenberg and Professor Rami Puzis, found that monitoring the sources of fake news, rather than each post or article, can significantly reduce the workload of fact-checkers and ensure consistent results over time.
“Today, the explosion of fake news is overwhelming fact-checkers. They cannot verify everything, which leads to uncertain coverage in the vast space of social networks and user notifications. In addition, there is limited understanding of how successful fact checkers are in identifying the most important information to check This prompted us to create a machine learning tool designed to help fact-checkers focus more effectively and improve their performance,” explains Dr. Greenberg. Their research was recently published in the Proceedings of the 30th ACM SIGKDD Conference on Knowledge Discovery and Data Mining.
Fake news sources tend to come and go quickly, making it expensive and time-consuming to maintain comprehensive lists of sites. The team’s system analyzes the flow of information on social platforms and measures the audience’s willingness to receive false content, which helps identify more sources and remains effective over time. Their audience-centric model significantly outperformed the conventional method of tracking misinformation sharers, with a 33% improvement with historical data and a 69% increase when identifying new sources.
The authors also demonstrated that their method can maintain the same level of accuracy in identifying fake news sources while requiring less than a quarter of the resources of conventional fact-checkers. While the system needs further testing in practical settings and should not replace human fact-checkers, “it can greatly enhance the capabilities of today’s fact-checkers,” says Dr. Greenberg, of the Department of Software Development and Information Systems. Professor Puzis is also a member of this department.
While Greenberg and his team have shown how this method can help fact-checkers protect the integrity of elections, a major unanswered question is whether social media companies will rise to the challenge, or at least provide the necessary data and access for others to combat disinformation.
Categories
- AI Education (39)
- AI in Business (65)
- AI Projects (87)
- Research (67)
- Uncategorized (3)
Other posts
- Breast Lift with Implants Abroad: Get the Perfect Shape with Trusted Clinics
- Find Best Clinic Abroad for Your Dental Treatment
- Discover World-Class Medical Care with Best Clinic Abroad
- Find the Best Medical Clinics Abroad: Quality Healthcare at Your Fingertips
- Why BestClinicAbroad.com is Your Ultimate Destination for Affordable, High-Quality Medical Treatment
- Discover World-Class Healthcare with BestClinicAbroad.com
- Get the Best Medical Treatment Abroad: A Complete Guide
- TOP Quality Medical Care with Best Clinic Abroad
- Discover World-Class Healthcare with Best Clinic Abroad
- Discover World-Class Healthcare Options with BestClinicAbroad.com
Newsletter
Get regular updates on data science, artificial intelligence, machine