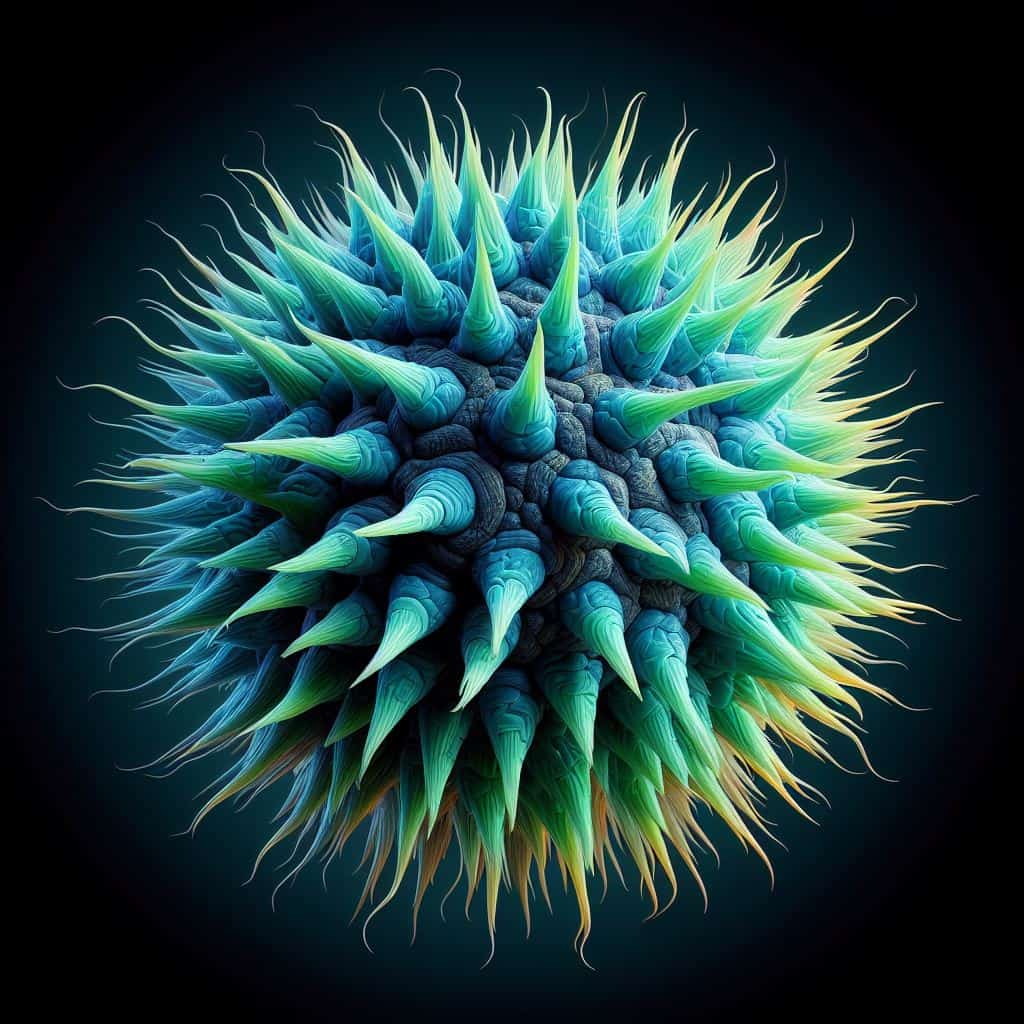
- December 29, 2023
- allix
- Research
Researchers at MIT, utilizing deep learning, have unearthed a novel class of compounds with the potential to combat a drug-resistant bacterium responsible for over 10,000 annual deaths in the United States.
Published in Nature, the study highlights the effectiveness of these compounds against methicillin-resistant Staphylococcus aureus (MRSA) in laboratory settings and two MRSA-infected mouse models. Significantly, these compounds demonstrate minimal toxicity to human cells, making them promising candidates for drug development.
An innovative aspect of the research lies in the researchers’ ability to unveil the information used by the deep-learning model to predict antibiotic potency. This newfound knowledge could facilitate the design of improved drugs surpassing those initially identified by the model.
James Collins, the Termeer Professor of Medical Engineering and Science at MIT’s Institute for Medical Engineering and Science (IMES) and Department of Biological Engineering, emphasizes the study’s efficiency and mechanistic insights, stating, “Our work provides a framework that is time-efficient, resource-efficient, and mechanistically insightful, from a chemical-structure standpoint, in ways that we haven’t had to date.”
Lead authors Felix Wong and Erica Zheng, part of the Antibiotics-AI Project at MIT, aim to discover new antibiotic classes targeting seven deadly bacteria over a seven-year period.
The study focuses on MRSA, which annually infects more than 80,000 people in the United States, causing various ailments, including skin infections and pneumonia. Severe cases can escalate to sepsis, a potentially life-threatening condition.
In recent years, MIT researchers, collaborating with the Abdul Latif Jameel Clinic for Machine Learning in Health, have utilized deep learning to identify potential antibiotics against various drug-resistant bacteria.
However, a challenge with this approach is the lack of transparency in the models, often referred to as “black boxes.” The study addresses this limitation by employing an algorithm called Monte Carlo tree search to decipher the model’s predictions, shedding light on the features influencing its decision-making.
The researchers expanded their training datasets, testing approximately 39,000 compounds for antibiotic activity against MRSA. By integrating information on chemical structures, they trained the deep learning model to predict antimicrobial activity.
To refine the selection of candidate drugs, the researchers employed three additional deep learning models to assess the compounds’ toxicity to human cells. The combination of antimicrobial predictions and toxicity assessments led to the identification of compounds effective against MRSA while posing minimal harm to human cells.
Screening around 12 million commercially available compounds, the models pinpointed five different classes with predicted activity against MRSA based on chemical substructures. After purchasing and testing approximately 280 compounds, two promising antibiotic candidates emerged from the same class.
These compounds, tested in two mouse models, demonstrated a significant reduction in MRSA populations, showcasing their potential as effective antibiotics. Further experiments indicated that the compounds disrupt bacteria by interfering with their ability to maintain an electrochemical gradient across cell membranes.
The researchers have shared their findings with Phare Bio, a nonprofit associated with the Antibiotics-AI Project, for in-depth analysis of the compounds’ properties and potential clinical use. Simultaneously, Collins’ lab is working on designing additional drug candidates based on the study’s insights.
In collaboration with institutions such as Harvard, the Broad Institute, Integrated Biosciences, Inc., the Wyss Institute for Biologically Inspired Engineering, and the Leibniz Institute of Polymer Research in Dresden, Germany, the research received funding from various sources, including the James S. McDonnell Foundation, the U.S. National Institute of Allergy and Infectious Diseases, and the Broad Institute.
The Antibiotics-AI Project, spearheaded by Collins, is funded by the Audacious Project, Flu Lab, the Sea Grape Foundation, the Wyss Foundation, and an anonymous donor.
Categories
- AI Education (33)
- AI in Business (58)
- AI Projects (80)
- Research (53)
Other posts
- Meta Introduced Llama 3 LLM
- Caffe – A Deep Learning Powerhouse
- The Study Reveals The Potential Of Artificial Intelligence To Take Over Key Management Functions In Scientific Research
- eBay Introduces New AI-Driven ‘Shop the Look’ Functionality to Its iOS Application
- Brave Releases Ai Assistant for iPhone and iPad Users
- Introduction to MXNet
- The Quiet-STAR Algorithm, Which Allows Chatbots To Think About Answers Before Responding
- Orchard’s Vision System Transforms Agricultural Machinery Into Ai-Powered Data Collection Tools
- Deepmind Introduces Genie – An Artificial Intelligence That Creates 2d Game Environments From A Single Image
- Microsoft Cognitive Toolkit (CNTK)
Newsletter
Get regular updates on data science, artificial intelligence, machine