A Fusion of Domain-Specific Assistance and Robust Language Models in an Embodied Conversational Agent
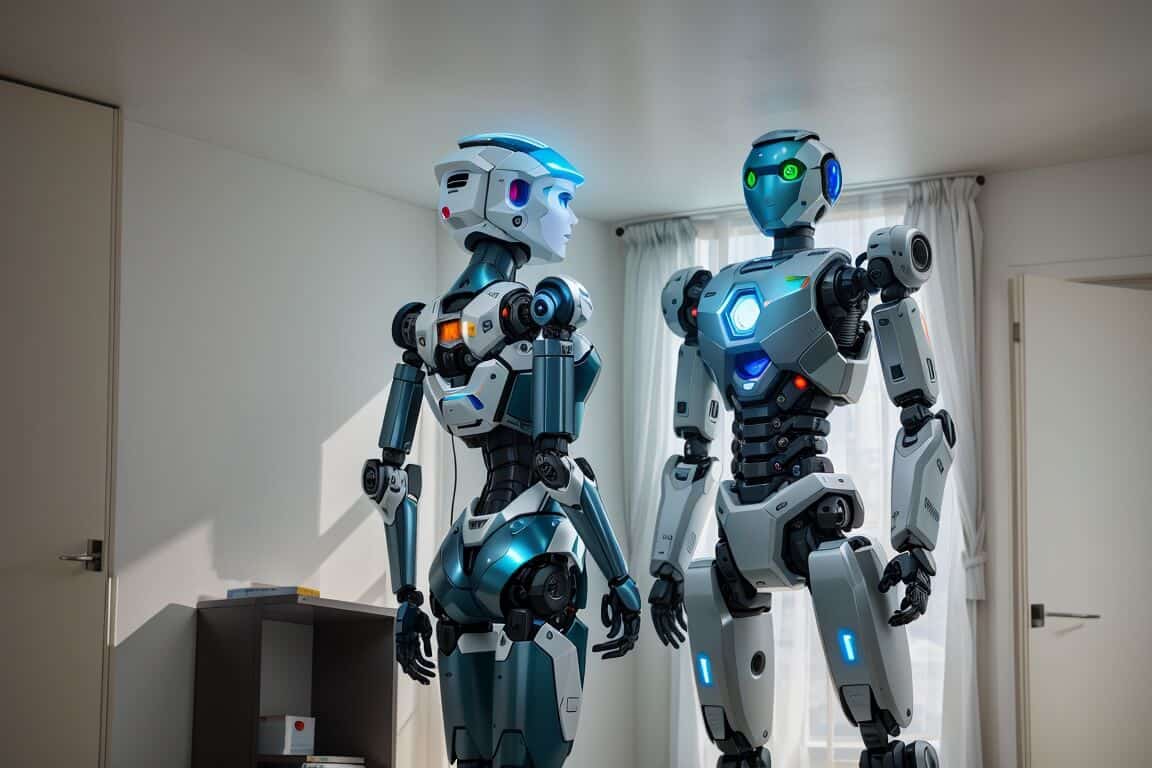
- September 13, 2023
- allix
- AI Projects
The large language models LLMs possess the capability to engage with humans in real-time, offering insightful responses to a wide array of queries. Their surge in popularity came about with the advent of ChatGPT, an OpenAI creation that astounded users with its capacity to generate human-like responses.
Despite their widespread adoption, most LLMs remain generic in nature, lacking the fine-tuning necessary to provide precise information within specific domains. Contrastingly, chatbots and robots deployed in locales like airports, shopping malls, and public spaces predominantly rely on distinct forms of natural language processing (NLP) models.
A collaborative effort between researchers at Heriot-Watt University and Alana AI gave birth to FurChat—an embodied conversational agent rooted in LLM technology, customized to offer context-specific information. This innovative agent, detailed in a pre-published paper on arXiv, engages users in lively spoken dialogues through the utilization of the Furhat robot, an anthropomorphic robotic head.
Oliver Lemon, one of the project’s researchers, explained, “Our aim was to explore various facets of embodied AI for natural human interaction. We were particularly interested in amalgamating the broad ‘open domain’ conversations facilitated by LLMs like ChatGPT with the provision of specialized and valuable information, such as details about a specific building or organization (in our case, the UK National Robotarium). We’ve also developed a similar system for dispensing information about a hospital (the Broca hospital in Paris for the SPRING project) using an ARI robot and in the French language.”
The primary goal of their recent work was to tailor LLMs to context-specific discussions. Lemon and his team sought to assess these models’ capacity to generate appropriate facial expressions in alignment with the content a robot or avatar conveys during interactions.
Lemon elucidated, “FurChat integrates a robust language model (LLM), such as ChatGPT or one of the numerous open-source alternatives like LLAMA, with a dynamically animated speech-enabled robot. To the best of our knowledge, it is the first system that seamlessly combines LLMs for both general conversation and specific information sourcing (e.g., data from organizational documents) with automated expressive robot animations.”
The responses and facial expressions of their embodied conversational agent emanate from the GPT 3.5 model and are subsequently conveyed verbally and physically through the Furhat robot.
To assess FurChat’s effectiveness, the researchers conducted a user evaluation, soliciting feedback from individuals who had interacted with the agent. Notably, they deployed the robot at the UK National Robotarium in Scotland, where it interacted with visitors, providing insights about the facility, ongoing research endeavors, upcoming events, and more.
Lemon elucidated further, stating, “We are delving into harnessing and advancing recent AI breakthroughs in LLMs to create systems that are not only more useful and user-friendly but also engrossing in human-robot-AI collaboration scenarios. Such systems must maintain a high level of factual accuracy, meticulously explaining the sources of their information, whether in documents or images.
“We are actively developing these features to ensure AI and robotic systems are both reliable and transparent. Concurrently, we are exploring systems that unite vision and language for embodied agents capable of seamless collaboration with humans, a development that is expected to gain increasing significance in the years ahead as we witness the emergence of more human-AI collaborative systems.”
In their inaugural real-world experiment, the FurChat system proved highly effective, facilitating smooth and informative interactions with users. This research holds promise for the potential introduction of LLM-based embodied AI agents in public spaces, museums, festivals, and various other venues.
Lemon concluded by revealing their future plans, “Our current efforts involve extending embodied conversational agents to encompass ‘multi-party’ dialogues, where interactions involve several humans, such as in hospital visits with family members. Subsequently, we aim to broaden their utilization in scenarios where teams of robots and humans collaborate to tackle real-world challenges.”
Categories
- AI Education (37)
- AI in Business (62)
- AI Projects (85)
- Research (57)
Other posts
- The Complete List of 28 US AI Startups to Earn Over $100 Million in 2024
- Keras Model
- Scientists Develop AI Solution to Prevent Power Outages
- NBC Introduces AI-Powered Legendary Broadcaster for Olympic Games
- Runway Introduces Video AI Gen-3
- Horovod – Distributed Deep Learning with TensorFlow and PyTorch
- Research Reveals Contradictory Positions Of AI Models On Controversial Issues
- Using AI to Understand Dog Barking
- The Autobiographer App Uses Artificial Intelligence To Record Your Life Story
- Chainer – Dynamic Neural Networks For Efficient Deep Learning
Newsletter
Get regular updates on data science, artificial intelligence, machine