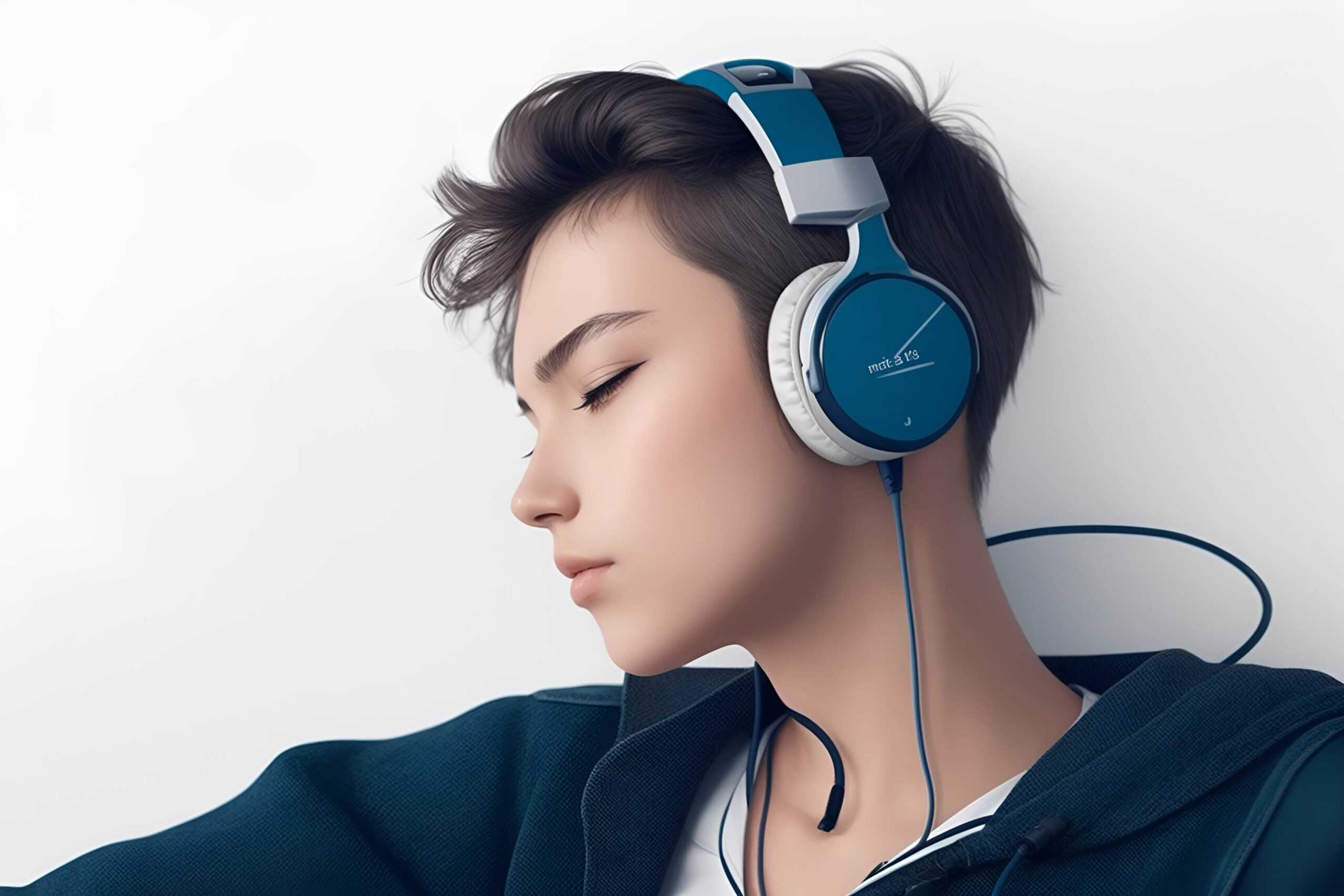
- November 10, 2023
- allix
- Research
Most individuals familiar with noise-canceling headphones understand the significance of filtering out specific sounds at specific moments. For instance, someone may desire to eliminate car honks while working indoors but not when strolling along bustling streets. Users typically lack the ability to choose which sounds their headphones cancel.
A group of researchers from the University of Washington, led by a team, has devised advanced deep-learning algorithms that empower users to actively select the sounds they want to pass through their headphones in real-time. This innovative system is termed “semantic hearing.” The headphones transmit recorded audio to a linked smartphone, which then cancels out all ambient sounds.
Through either vocal commands or a smartphone application, headphone users can handpick the sounds they wish to include from a list of 20 categories, including sirens, baby cries, speech, vacuum cleaners, and bird chirps. Only the chosen sounds will be relayed through the headphones.
The team unveiled their discoveries on November 1 at UIST ’23 in San Francisco, with future plans to introduce a commercial version of the system.
Senior author Shyam Gollakota, a professor in the Paul G. Allen School of Computer Science & Engineering at UW, emphasized the real-time intelligence required to discern and extract specific sounds from the surrounding environment. He explained that existing noise-canceling headphones face challenges in synchronizing the sounds with users’ visual senses, necessitating neural algorithms capable of processing sounds within a hundredth of a second.
Due to this time constraint, the semantic hearing system processes sounds on a device like a connected smartphone, rather than relying on more powerful cloud servers. The system must preserve the temporal delays and spatial cues associated with sounds arriving from different directions, ensuring users can still perceptively engage with their surroundings.
Tested in various settings, such as offices, streets, and parks, the system successfully isolated target sounds like sirens, bird chirps, and alarms while eliminating all other background noise. Participants, when rating the system’s audio output for the target sounds, reported an average improvement in quality compared to the original recordings.
The system faced challenges in distinguishing between sounds sharing similar properties, such as vocal music and human speech. The researchers suggest that refining the models through additional real-world data could enhance these outcomes. Co-authors on the paper include Bandhav Veluri and Malek Itani, both doctoral students at UW; Justin Chan, a former doctoral student at the Allen School now at Carnegie Mellon University; and Takuya Yoshioka, research director at AssemblyAI.
Categories
- AI Education (37)
- AI in Business (62)
- AI Projects (85)
- Research (57)
Other posts
- The Complete List of 28 US AI Startups to Earn Over $100 Million in 2024
- Keras Model
- Scientists Develop AI Solution to Prevent Power Outages
- NBC Introduces AI-Powered Legendary Broadcaster for Olympic Games
- Runway Introduces Video AI Gen-3
- Horovod – Distributed Deep Learning with TensorFlow and PyTorch
- Research Reveals Contradictory Positions Of AI Models On Controversial Issues
- Using AI to Understand Dog Barking
- The Autobiographer App Uses Artificial Intelligence To Record Your Life Story
- Chainer – Dynamic Neural Networks For Efficient Deep Learning
Newsletter
Get regular updates on data science, artificial intelligence, machine