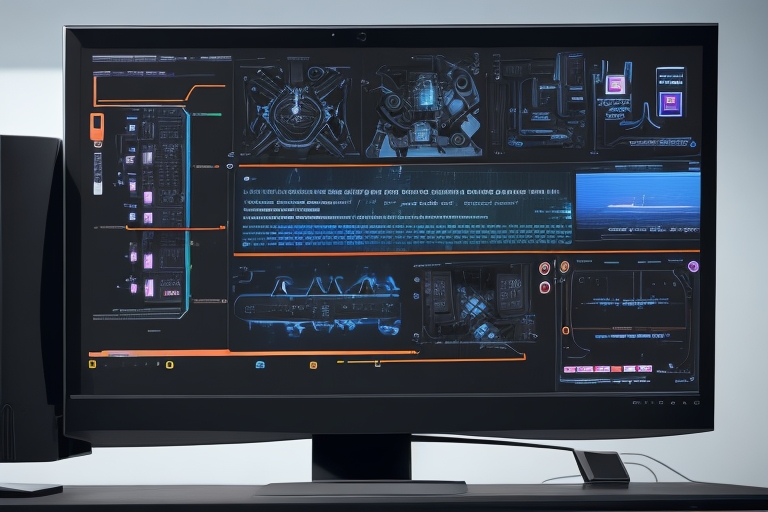
- November 16, 2022
- allix
- Research
Large Language Models (LLM) have a well-kept secret: their development and operation require large amounts of energy. Furthermore, the true extent of the carbon footprint of these models remains a mystery. The start-up Hugging Face thinks it has found a way to calculate this footprint more accurately by estimating the emissions produced during the entire life cycle of the model and not just during its development.
The attempt could be a step towards getting more realistic data from tech companies about the carbon footprint of their artificial intelligence (AI) products at a time when experts are calling on the industry to better assess the impact environment of AI. Hugging Face’s work is published in an article that has not yet been peer-reviewed.
In order to test its new approach, Hugging Face evaluated the global emissions of its own language model called BLOOM. The latter was launched at the beginning of the year. This intricate process entailed the accumulation of numerous numbers, encompassing a range of energy factors. From the energy expended during the model’s training on a supercomputer to the energy required for manufacturing the supercomputer’s hardware and sustaining its computing infrastructure, and finally, the energy consumption of BLOOM once it is fully deployed. The researchers calculated this last part using a software tool called CodeCarbon. This monitored the carbon emissions produced by BLOOM in real time over an 18-day period.
Hugging Face estimated that the development of BLOOM had caused the emission of 25 tons of carbon. The researchers made a significant revelation as they delved deeper into the analysis. The initial figure was doubled when they factored in the emissions arising from the manufacturing of the computer hardware utilized for the LLM’s development, along with the broader computing infrastructure. Additionally, the energy needed to sustain BLOOM once the development phase is completed also contributed to this revised assessment.
Helping the AI community get a better idea of its impact on the environment
While this number may seem high for a single model – 50 metric tons of carbon emissions or the equivalent of approximately 60 flights from London to New York – it is significantly lower than the emissions associated with other LLMs of the same size. The significant reduction in carbon emissions can be attributed to BLOOM’s development of a French supercomputer, predominantly powered by nuclear energy, which emits no carbon. Models formed in China, Australia, or parts of the United States, whose energy networks rely more on fossil fuels, are likely to be more polluting.
Following the successful launch of BLOOM, Hugging Face conducted estimations that indicated the model’s usage was associated with approximately 19 kilograms of carbon dioxide emissions per day. A number that is similar to the emissions produced by an average new car driven just over 85 kilometers.
For comparison, OpenAI’s GPT-3 and Meta’s OPT are estimated to emit more than 500 and 75 metric tons of carbon dioxide, respectively, during development. The extent of GPT-3 emissions can be partly explained by the fact that it was trained on older, less efficient hardware. But it is difficult to say with certainty about these figures. The measurement of carbon emissions in this context lacks a standardized approach, and the figures provided are based on external estimates or limited data made available by companies like Meta.
“Our goal was to go beyond just the carbon emissions of electricity consumed during development and consider more of the lifecycle to help the AI community have a better idea of its impact on the environment and how we can start to reduce that impact,” says Sasha Luccioni, a researcher at Hugging Face and lead author of the paper.
The carbon footprint of language models
According to Emma Strubell, an assistant professor at Carnegie Mellon University’s School of computer science, who previously authored a seminal article on the environmental impact of AI in 2019, the Hugging Face article has set a new standard for organizations involved in AI model development.
Emma Strubell emphasizes that this paper represents the most comprehensive, honest, and thoroughly researched analysis of the carbon footprint of a large machine learning (ML) model to date. As far as she knows, it surpasses any other article or report in terms of depth and detail.
According to Lynn Kaack, an assistant professor of computer science and public policy at the Hertie School in Berlin, who was not involved in Hugging Face’s work, the article also sheds much-needed light on the extent of the footprint. the carbon of large language models. She says she was surprised to see the scale of the life cycle emissions figures but feels there is still a lot to be done to understand the environmental impact of large language models in the real world.
“We need to better understand the far more complex downstream effects of AI use and abuse…It’s much harder to estimate. That’s why this part is often overlooked”, details Lynn Kaack. The latter co-wrote an article published last summer in Nature magazine which offered a way to measure on-chain emissions caused by AI systems.
The technology sector accounts for approximately 2% to 4% of global greenhouse gas emissions.
For example, recommendation and advertising algorithms are often used in advertising which in turn tricks people into consuming and buying more stuff resulting in more carbon emissions. According to Lynn Kaack, it is also important to understand how artificial intelligence models are employed. Many companies, such as Google and Meta, use AI models to rank user comments or recommend content to them. Taken individually, these actions consume very little energy, but given that they are carried out a billion times a day, they add up, and emissions increase.
It is estimated that the technology sector, as a whole, is responsible for 1.8% to 3.9% of global greenhouse gas emissions. Although AI and machine learning are only responsible for a fraction of these emissions, the carbon footprint of AI is still very high for a single technology sector.
Thanks to a better understanding of the amount of energy consumed by AI systems, companies and developers can make choices about the trade-offs they are willing to make between pollution and costs generated, analyzes Sasha Luccioni.
A “warning signal” for major technology groups
The authors of the Hugging Face article hope that companies and researchers will be able to think about how they can develop great language models while limiting their carbon footprint, says Sylvain Viguier, co-author of the article in question and director of applications at Graphcore, a semiconductor company.
It could also encourage people to move towards more efficient methods of AI research by, for example, refining existing models rather than pushing to create even larger models, adds Sasha Luccioni.
The conclusions of the article constitute “a warning sign for people who use this type of model, that is to say, most of the time people working for large technology companies”, considers David Rolnick, assistant professor at the computer science school of McGill University and at the Quebec Institute of Artificial Intelligence (Mila). With Lynn Kaack, he is one of the co-authors of the article published in Nature but he did not participate in the work of Hugging Face.
Categories
- AI Education (37)
- AI in Business (62)
- AI Projects (85)
- Research (57)
Other posts
- The Complete List of 28 US AI Startups to Earn Over $100 Million in 2024
- Keras Model
- Scientists Develop AI Solution to Prevent Power Outages
- NBC Introduces AI-Powered Legendary Broadcaster for Olympic Games
- Runway Introduces Video AI Gen-3
- Horovod – Distributed Deep Learning with TensorFlow and PyTorch
- Research Reveals Contradictory Positions Of AI Models On Controversial Issues
- Using AI to Understand Dog Barking
- The Autobiographer App Uses Artificial Intelligence To Record Your Life Story
- Chainer – Dynamic Neural Networks For Efficient Deep Learning
Newsletter
Get regular updates on data science, artificial intelligence, machine