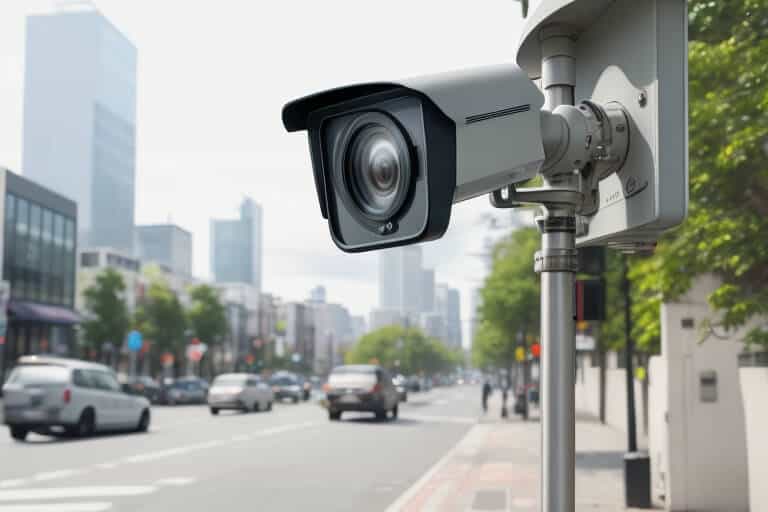
- September 28, 2023
- allix
- Research
While photographs are inherently two-dimensional (2D), the demands of autonomous vehicles and various technological applications require navigation within a three-dimensional (3D) environment. Enterprising researchers have devised a novel approach aimed at assisting artificial intelligence (AI) in extracting 3D insights from 2D images, thereby amplifying the utility of cameras for these burgeoning technologies.
“Present methods for extracting 3D information from 2D images are competent but not entirely satisfactory,” asserts Tianfu Wu, a co-author of the study and an associate professor of electrical and computer engineering at North Carolina State University. “Our fresh methodology, dubbed ‘MonoXiver,’ can be synergistically integrated with existing techniques, significantly enhancing their accuracy.”
This advancement holds particular promise for applications like autonomous vehicles, given the cost-effectiveness of cameras compared to alternative 3D spatial navigation tools such as LIDAR, which relies on laser technology for distance measurement. As cameras are more economically viable, designers of autonomous vehicles can deploy multiple cameras to build redundancy within the system.
The utility of this redundancy hinges on the ability of AI systems within autonomous vehicles to extract 3D navigation data from the 2D images captured by these cameras, and this is precisely where MonoXiver steps in.
Existing techniques that extract 3D data from 2D images, exemplified by the MonoCon method developed by Wu and his collaborators, make use of “bounding boxes.” These techniques train AI systems to scrutinize a 2D image and encase objects in the image with 3D bounding boxes, such as the vehicles on a street.
These bounding boxes take the form of cuboids, each defined by eight points, akin to the corners of a shoebox. These bounding boxes enable the AI to estimate the dimensions of objects within the image and their spatial relationships with other objects. They help the AI gauge the size and position of a car relative to other vehicles on the road.
Existing bounding box techniques often prove imperfect and fail to encompass certain parts of an object or vehicle depicted in a 2D image.
MonoXiver introduces a fresh approach by utilizing each bounding box as an initial reference point or anchor and then instructs the AI to conduct a secondary analysis of the area surrounding each bounding box. This secondary analysis generates numerous additional bounding boxes, all encompassing the anchor.
To discern which of these secondary boxes best captures any omitted portions of the object, the AI engages in two comparisons. One comparison examines the “geometry” of each secondary box to ascertain if it contains shapes consistent with those in the anchor box. The other comparison evaluates the “appearance” of each secondary box, checking for colors or visual characteristics that align with those found within the anchor box.
“One noteworthy leap forward with MonoXiver is its capacity to execute this top-down sampling technique—creating and analyzing secondary bounding boxes—exceptionally efficiently,” Wu remarks.
To gauge the effectiveness of the MonoXiver approach, researchers tested it using two datasets of 2D images: the established KITTI dataset and the more challenging, extensive Waymo dataset.
“We employed the MonoXiver technique in conjunction with MonoCon and two other existing programs designed for extracting 3D data from 2D images, and MonoXiver noticeably elevated the performance of all three programs,” Wu affirms. “The most impressive results were achieved when MonoXiver was employed in tandem with MonoCon.”
“It’s crucial to note that this enhancement comes with only minor computational overhead,” Wu adds. “For instance, MonoCon, in isolation, operates at 55 frames per second. When incorporating the MonoXiver method, this rate slightly reduces to 40 frames per second, which still remains sufficiently swift for practical applications.”
“We are enthusiastic about this breakthrough and will continue refining and evaluating it for implementation in autonomous vehicles and various other domains,” Wu concludes.
Categories
- AI Education (39)
- AI in Business (65)
- AI Projects (87)
- Research (72)
- Uncategorized (3)
Other posts
- Buccal Fat Extraction: Sculpt Your Dream Look with the Experts at Best Clinic Abroad
- Get Fast Relief from Spinal Fractures: Kyphoplasty Surgery Abroad with BestClinicAbroad
- Curious About Genetic Testing for Fertility? Here’s What You Need to Know (and Why People Trust Best Clinic Abroad to Book It)
- Hip Pain Ruining Your Life? Here’s Why More People Are Getting Hip Replacements Abroad with Best Clinic Abroad
- Breathe Easier: Why Best Clinic Abroad is Your Top Choice for Septoplasty
- Breast Lift with Implants Abroad: Get the Perfect Shape with Trusted Clinics
- Find Best Clinic Abroad for Your Dental Treatment
- Discover World-Class Medical Care with Best Clinic Abroad
- Find the Best Medical Clinics Abroad: Quality Healthcare at Your Fingertips
- Why BestClinicAbroad.com is Your Ultimate Destination for Affordable, High-Quality Medical Treatment
Newsletter
Get regular updates on data science, artificial intelligence, machine