Nanoelectronic Device Empowers Real-Time AI Classification Independently of Cloud Services
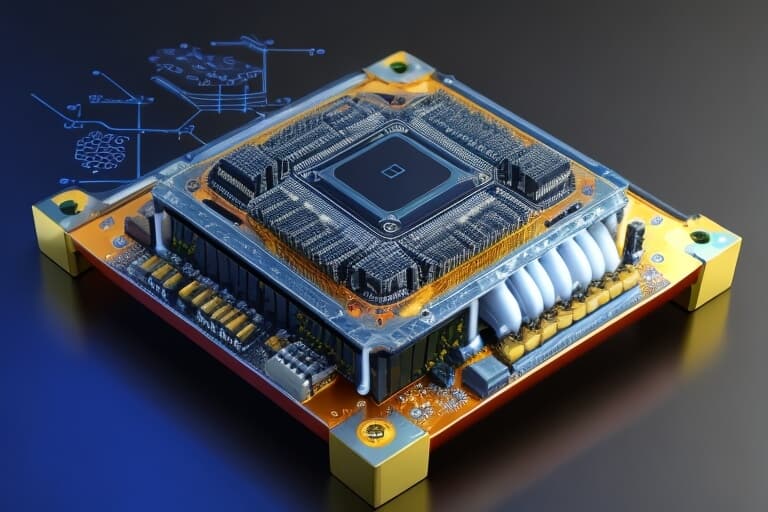
- October 12, 2023
- allix
- AI Projects
Leave behind the cloud, as Northwestern University engineers have introduced a cutting-edge nanoelectronic device capable of executing precise machine-learning classification tasks in an extraordinarily energy-efficient manner. Consuming a mere 100-fold less energy than contemporary technologies, this device can effortlessly process substantial volumes of data and perform real-time artificial intelligence (AI) operations without the need to transmit data to the cloud for analysis.
This diminutive device, characterized by its minimal power consumption and swift response time, is perfectly suited for seamless integration into wearable electronics, such as smartwatches and fitness trackers. This enables real-time data processing and almost instant diagnostic capabilities.
To validate their concept, engineers employed the device to categorize extensive data from publicly available electrocardiogram (ECG) datasets. Impressively, the device not only proficiently and accurately identified irregular heartbeats but also distinguished among six distinct arrhythmia subtypes with nearly 95% precision.
Their study, titled “Reconfigurable mixed-kernel heterojunction transistors for personalized support vector machine classification,” was published in the journal Nature Electronics on October 12.
Mark C. Hersam, the lead researcher behind this study and a distinguished specialist in the field of nanotechnology at Northwestern University, expressed, “In the present scenario, the majority of sensors amass data and subsequently transmit it to the cloud, where the analysis takes place on power-intensive servers before the findings are eventually relayed back to the user. This methodology is exceedingly costly, exerts a substantial energy demand, and introduces time delays. Our device, with its exceptional energy efficiency, can be seamlessly integrated into wearable electronics for instantaneous data detection and processing, facilitating swifter responses during health emergencies.”
Hersam, the Walter P. Murphy Professor of Materials Science and Engineering at Northwestern’s McCormick School of Engineering, and his collaborators, including Han Wang, a professor at the University of Southern California, and Vinod Sangwan, a research assistant professor at Northwestern, co-led this groundbreaking research.
Before machine-learning tools can analyze new data, they must proficiently categorize training data into various segments. For instance, when classifying photos by color, the tool must accurately recognize which photos are red, yellow, or blue to categorize them correctly. While a simple task for a human, this is a complex and energy-intensive undertaking for a machine.
With current silicon-based technologies, classifying data from extensive datasets like ECGs demands over 100 transistors, each requiring its own power supply. Northwestern’s nanoelectronic device accomplishes the same machine-learning classification task with just two devices. This substantial reduction in the number of devices considerably decreases power consumption and results in a much smaller device suitable for integration into typical wearable gadgets.
The core innovation behind this novel device lies in its exceptional tunability, stemming from a blend of materials. Unlike traditional technologies that rely on silicon, the researchers constructed miniaturized transistors using two-dimensional molybdenum disulfide and one-dimensional carbon nanotubes. This approach eliminates the need for numerous silicon transistors, as each can dynamically switch between various processing steps.
Hersam elaborated, stating, “Combining two distinct materials within a single device grants us the capability to effectively control the current flow by applying voltages, resulting in dynamic adaptability. This heightened level of adjustability within a single device empowers us to execute complex classification algorithms with a compact design and minimal energy usage.”
In their testing, the researchers employed publicly available medical datasets. Initially, they trained the device to interpret ECG data, a task that typically consumes significant time from trained healthcare professionals. Subsequently, they tasked the device with classifying six types of heartbeats: normal, atrial premature beat, premature ventricular contraction, paced beat, left bundle branch block beat, and right bundle branch block beat.
The nanoelectronic device accurately identified each arrhythmia type out of 10,000 ECG samples. Bypassing the necessity to transmit data to the cloud not only saves crucial time for patients but also enhances data privacy.
Hersam underscored the advantages for data security, explaining, “Whenever data is transmitted, the potential for data breaches escalates. Processing personal health data at a local level, such as within your wrist-worn device, substantially diminishes security risks. In this way, our device enhances privacy and diminishes the susceptibility to breaches.”
Hersam envisions that, in the future, these nanoelectronic devices could seamlessly integrate into everyday wearables, tailoring themselves to each user’s unique health profile for real-time applications. This would allow people to harness the data they collect without draining power resources.
Hersam concluded, “Artificial intelligence tools are consuming an increasing fraction of the power grid. It is an unsustainable path if we continue relying on conventional computer hardware.”
Categories
- AI Education (39)
- AI in Business (65)
- AI Projects (87)
- Research (79)
- Uncategorized (3)
Other posts
- Advanced Cancer & Blood Disease Treatment Abroad: Hope, Expertise, and Global Care 🌍🩺
- Healthcare in the UAE: Premium Treatment, World-Class Clinics, and No Waiting Time
- Neck Liposuction Abroad: Say Goodbye to Double Chin, Hello to Defined Confidence
- Dental Veneers Abroad: Get Your Dream Smile for Less
- Endoscopic Brain Surgery Abroad: Safe, Affordable & Advanced Options You Can Trust
- Say Goodbye to Unwanted Moles: Discover Safe, Affordable Mole Removal Abroad
- Mastoidectomy Abroad: Affordable, Safe, and Life-Changing Ear Surgery
- Buccal Fat Extraction: Sculpt Your Dream Look with the Experts at Best Clinic Abroad
- Get Fast Relief from Spinal Fractures: Kyphoplasty Surgery Abroad with BestClinicAbroad
- Curious About Genetic Testing for Fertility? Here’s What You Need to Know (and Why People Trust Best Clinic Abroad to Book It)
Newsletter
Get regular updates on data science, artificial intelligence, machine