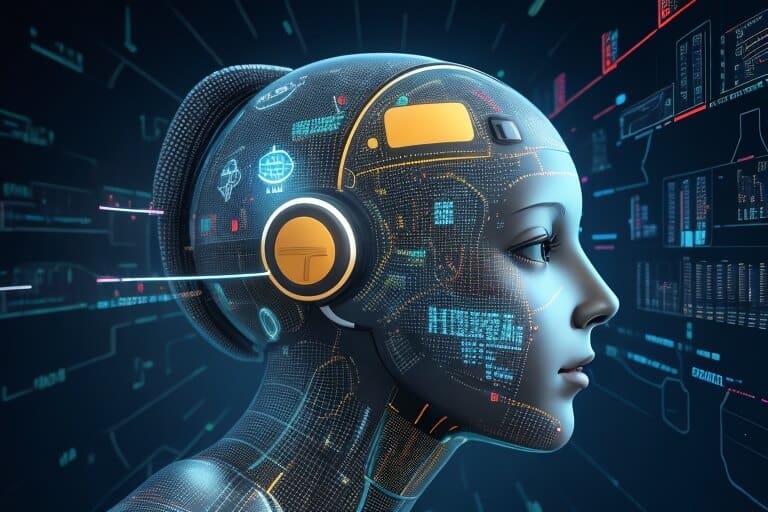
- November 1, 2023
- allix
- Research
Professor Sang-hyun Park’s research team at the Department of Robotics and Mechatronics Engineering, Daegu Gyeongbuk Institute of Science and Technology (DGIST), has introduced a novel image translation model with the potential to mitigate biases in data effectively.
When constructing artificial intelligence (AI) models using images gathered from diverse sources, data biases can inadvertently emerge due to various factors, even when the user’s intent is to avoid them. This newly developed model can rectify data biases without the need for specific knowledge about these factors, leading to superior image analysis performance. This breakthrough is poised to foster innovations in self-driving technology, content creation, and healthcare.
Datasets employed in training deep learning models often exhibit biases. For instance, while assembling a dataset to differentiate bacterial pneumonia from COVID-19, variations in image collection conditions may arise due to COVID-19-related concerns. These variations introduce subtle discrepancies in the images, causing existing deep learning models to classify diseases based on image protocol variations rather than the vital characteristics for practical disease identification.
These models excel when evaluated with data employed during their training process but falter when dealing with data from different sources, as they struggle to generalize effectively, leading to over-fitting problems. Existing deep learning methods tend to rely on textural differences as crucial data, which can result in inaccurate predictions.
To tackle these issues, Professor Park’s research team devised an image translation model that generates a dataset while mitigating textural biases and leverages this dataset for the learning process.
This new image translation model operates by extracting information regarding an input image’s content and textures from a distinct domain and combining them.
To preserve information about both the input images’ content and the texture of the new domain, the model is trained using error functions for spatial self-similarity and texture co-occurrence. Through these processes, the model can produce an image with the texture of a different domain while retaining the input image’s content information.
Because the developed deep learning model generates a dataset while mitigating texture biases and employs this dataset for training, it outperforms existing models in terms of performance.
When tested on datasets exhibiting texture biases, such as classification datasets distinguishing numbers, dogs, cats with varying coat colors, and COVID-19 from bacterial pneumonia with different image protocols, it consistently delivered superior results compared to existing debiasing and image translation methods. It excelled when applied to datasets featuring diverse biases, such as classification datasets for multi-label numbers, photos, images, animations, and sketches.
Professor Park’s image translation technology can be applied to image manipulation. The research team found that their method altered only the image’s textures while preserving its original content. This analysis affirmed the method’s superior performance compared to existing image manipulation techniques.
This solution can be effectively employed in various domains. The research team compared its performance with existing image translation methods across domains like medicine and self-driving images and found it consistently outperformed existing methods.
Professor Park noted, “The technology developed in this research significantly enhances performance when dealing with biased datasets, which are inevitable in the training of deep learning models for industrial and medical applications.”
He added, “This solution is poised to make a substantial contribution to bolstering the resilience of AI models deployed in diverse commercial environments.”
Categories
- AI Education (39)
- AI in Business (65)
- AI Projects (87)
- Research (72)
- Uncategorized (3)
Other posts
- Buccal Fat Extraction: Sculpt Your Dream Look with the Experts at Best Clinic Abroad
- Get Fast Relief from Spinal Fractures: Kyphoplasty Surgery Abroad with BestClinicAbroad
- Curious About Genetic Testing for Fertility? Here’s What You Need to Know (and Why People Trust Best Clinic Abroad to Book It)
- Hip Pain Ruining Your Life? Here’s Why More People Are Getting Hip Replacements Abroad with Best Clinic Abroad
- Breathe Easier: Why Best Clinic Abroad is Your Top Choice for Septoplasty
- Breast Lift with Implants Abroad: Get the Perfect Shape with Trusted Clinics
- Find Best Clinic Abroad for Your Dental Treatment
- Discover World-Class Medical Care with Best Clinic Abroad
- Find the Best Medical Clinics Abroad: Quality Healthcare at Your Fingertips
- Why BestClinicAbroad.com is Your Ultimate Destination for Affordable, High-Quality Medical Treatment
Newsletter
Get regular updates on data science, artificial intelligence, machine