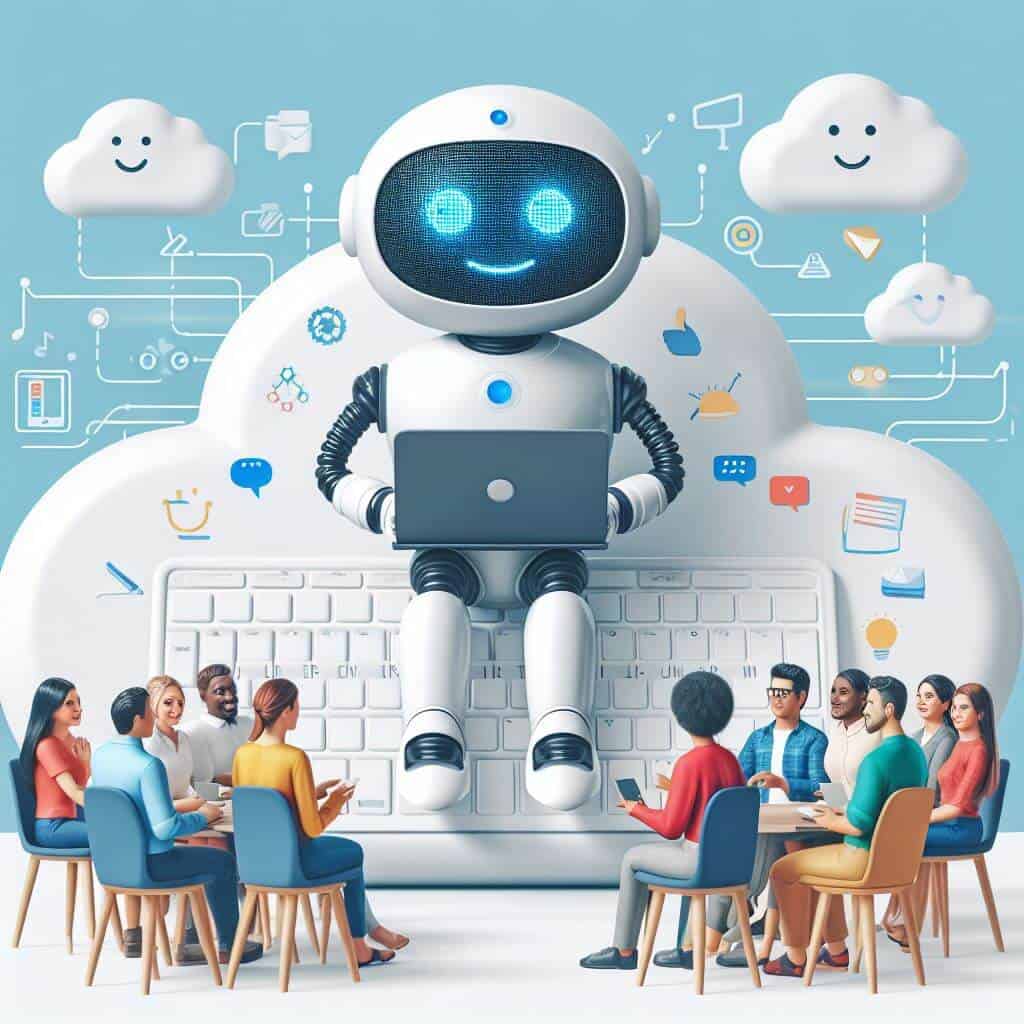
- May 24, 2024
- allix
- Research
A team of artificial intelligence experts at AWS AI Labs, part of Amazon, discovered that almost all publicly available large language models (LLMs) can be easily manipulated to reveal dangerous or unethical information.
In their article on the arXiv preprint server, the researchers explain how they discovered that LLMs like ChatGPT can be tricked into providing unauthorized responses and suggest a solution to the problem. When LLMs became available to the public, it quickly became apparent that many people were using them for malicious purposes. This included receiving instructions on illegal activities such as making explosives, tax evasion, or committing robberies. Others have abused this technology to create and distribute hateful content online.
In response, the developers of these systems began implementing rules to prevent LLM from responding to potentially dangerous, illegal, or malicious requests. In this new study, AWS researchers determined that such measures are not sufficient, as they can be easily circumvented with simple beeps. The team’s research involved breaking through the defenses of several LLMs by adding audio elements during the queries, which helped them avoid limitations imposed by the modelers. While the researchers refrain from giving specific examples to prevent misuse, they do mention using a technique called predictive gradient descent.
As an indirect illustration, they discuss how using basic assertions with one model, followed by an original question, can cause the model to ignore its limitations. Researchers have noted varying degrees of success in bypassing different LLMs, depending on their level of access to the model. They also noticed that techniques that worked on one model often worked on others. Finally, the team suggests that the LLM developers could strengthen the security mechanisms by adding elements such as random noise to the audio inputs, making it more difficult for users to bypass the security measures.
Categories
- AI Education (37)
- AI in Business (62)
- AI Projects (85)
- Research (57)
Other posts
- The Complete List of 28 US AI Startups to Earn Over $100 Million in 2024
- Keras Model
- Scientists Develop AI Solution to Prevent Power Outages
- NBC Introduces AI-Powered Legendary Broadcaster for Olympic Games
- Runway Introduces Video AI Gen-3
- Horovod – Distributed Deep Learning with TensorFlow and PyTorch
- Research Reveals Contradictory Positions Of AI Models On Controversial Issues
- Using AI to Understand Dog Barking
- The Autobiographer App Uses Artificial Intelligence To Record Your Life Story
- Chainer – Dynamic Neural Networks For Efficient Deep Learning
Newsletter
Get regular updates on data science, artificial intelligence, machine