Revolutionizing Content Discovery Through Recommendation Systems
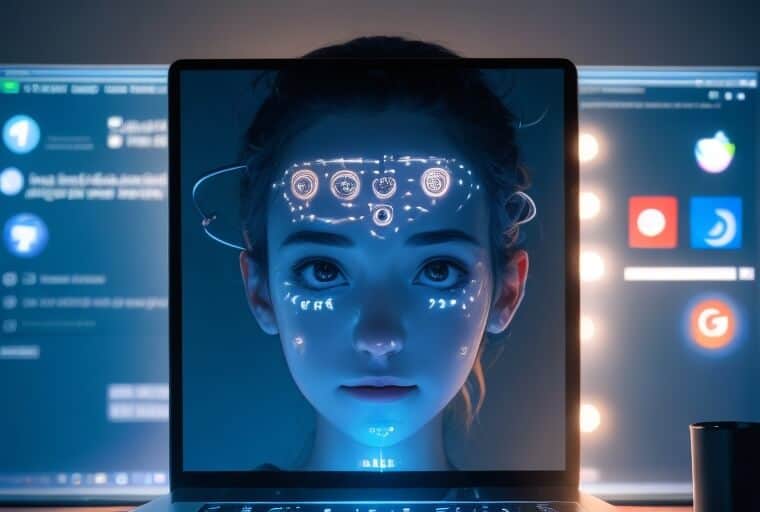
- September 5, 2023
- allix
- AI in Business
Artificial Intelligence (AI) has woven its intricate threads into nearly every aspect of our daily lives, and the world of entertainment is no exception. In recent years, AI-powered recommendation systems have emerged as a game-changer in how we discover and consume entertainment content. These systems employ sophisticated algorithms and machine learning techniques to tailor content recommendations to individual preferences, reshaping the entertainment industry landscape.
The Evolution of Recommendation Systems
The start of recommendation systems in entertainment can be traced back to the early 2000s when trailblazing companies like Netflix and Amazon began experimenting with personalized content suggestions. These companies harnessed the power of collaborative filtering techniques, which analyzed user behavior and preferences to recommend movies, books, and products. Although relatively rudimentary by today’s standards, these early systems laid the foundation for the AI-driven recommendation engines we witness today.
Collaborative filtering, in its various forms, remains a foundational aspect of modern recommendation systems. This approach operates on the simple yet effective principle that users who have exhibited similar preferences in the past will likely continue to do so in the future. Collaborative filtering can be further divided into two main approaches: user-based and item-based.
User-based collaborative filtering involves comparing the historical interactions of users and recommending items that users with similar behavior have shown appreciation for. In contrast, item-based collaborative filtering takes a different route by analyzing the similarity between items and suggesting those that bear likeness to those a user has previously shown interest in. These techniques laid the groundwork for more advanced AI-driven algorithms, ensuring that recommendations became increasingly precise and tailored.
As the realm of AI and machine learning advanced, recommendation systems evolved beyond the confines of simple collaborative filtering. Machine learning algorithms, such as decision trees, random forests, and gradient boosting, were enlisted to capture complex patterns in user behavior. These models factored in a plethora of features, ranging from user demographics and viewing history to real-time context, enabling more refined content recommendations.
In recent years, deep learning has taken center stage in recommendation systems. Deep neural networks, particularly variants like recurrent neural networks (RNNs) and convolutional neural networks (CNNs), have demonstrated remarkable capabilities in capturing intricate user preferences. These neural networks excel in handling unstructured data such as textual reviews, image metadata, and even audio sentiment analysis, enriching content recommendations with a deeper understanding of user intent.
The Impact on Entertainment
The incorporation of AI-driven recommendation systems in the entertainment industry has been transformative in several key ways. One of the most profound impacts of recommendation systems is the unparalleled level of personalization they bring to the entertainment experience. By scrutinizing user data, these systems can provide tailored content suggestions, ensuring that viewers are more likely to engage with the content presented to them. For example, when a streaming service like Spotify recommends music tracks based on a user’s listening history and preferences, it enhances the user’s engagement and enjoyment.
This personal touch has led to increased user engagement, longer viewing times, and improved customer satisfaction. Streaming giants like Netflix have leveraged this by adapting their interfaces to prominently feature personalized recommendations on users’ homepages, contributing significantly to their continued growth and subscriber retention.
In the vast landscape of entertainment, discovering new and relevant content can be akin to finding a needle in a haystack. Recommendation systems address this challenge by helping users uncover hidden gems and explore content outside their comfort zone. This not only benefits consumers but also content creators and platforms.
For instance, YouTube’s recommendation algorithm uses a combination of collaborative filtering and deep learning to suggest videos related to a user’s viewing history. It goes a step further by introducing serendipity into the mix, offering videos from diverse genres that align with the user’s interests but also introducing them to fresh content they might not have discovered otherwise. This balanced approach fosters a richer and more diverse content ecosystem.
For streaming platforms and content providers, the implementation of recommendation systems has proven to be a substantial revenue driver. By keeping viewers engaged and offering content they are more likely to enjoy, these systems increase subscription retention rates and facilitate targeted advertising.
Consider Amazon’s recommendation engine. It not only suggests products but also aligns them with a user’s browsing and purchasing history. This targeted approach leads to higher conversion rates, maximizing revenue. In the realm of streaming, Hulu employs AI to customize advertising, ensuring that viewers are presented with ads that align with their interests, making them more likely to engage with the advertisements.
Challenges in Recommendation Systems
While AI-powered recommendation systems have come a long way, they still grapple with several challenges that must be addressed to provide even better user experiences. The cold start problem arises when a recommendation system has limited or no data about a new user. Without sufficient information, it’s challenging to make accurate recommendations. To mitigate this issue, hybrid recommendation systems combine collaborative filtering, content-based filtering, and other techniques to provide initial suggestions.
For instance, when a user signs up for a streaming service like Disney+, the system may initially recommend popular or trending content to engage the user. Over time, as the system collects data on the user’s viewing habits and preferences, recommendations become increasingly personalized.
Balancing accuracy and serendipity in recommendations is an ongoing challenge. While users appreciate content that aligns with their preferences, they also seek surprises and diversity in their entertainment choices. Striking the right balance is essential for maintaining user engagement.
Netflix is a prime example of a platform that seeks this balance. Its recommendation engine, powered by a blend of collaborative filtering and deep learning, recommends content that aligns with a user’s past choices but also includes a “Recommended for You” section that introduces users to unexpected gems, expanding their viewing horizons.
The collection and utilization of user data raise significant concerns about privacy and ethics. Striking a balance between providing personalized recommendations and respecting user privacy is a delicate task. AI developers must prioritize data security and transparent data usage policies.
In recent years, there have been cases where data misuse has led to public outcry. Companies like Facebook have faced scrutiny over how they use user data for ad targeting. To address these concerns, the entertainment industry is gradually moving toward more transparent data handling practices and giving users greater control over their data.
Categories
- AI Education (37)
- AI in Business (62)
- AI Projects (85)
- Research (57)
Other posts
- The Complete List of 28 US AI Startups to Earn Over $100 Million in 2024
- Keras Model
- Scientists Develop AI Solution to Prevent Power Outages
- NBC Introduces AI-Powered Legendary Broadcaster for Olympic Games
- Runway Introduces Video AI Gen-3
- Horovod – Distributed Deep Learning with TensorFlow and PyTorch
- Research Reveals Contradictory Positions Of AI Models On Controversial Issues
- Using AI to Understand Dog Barking
- The Autobiographer App Uses Artificial Intelligence To Record Your Life Story
- Chainer – Dynamic Neural Networks For Efficient Deep Learning
Newsletter
Get regular updates on data science, artificial intelligence, machine