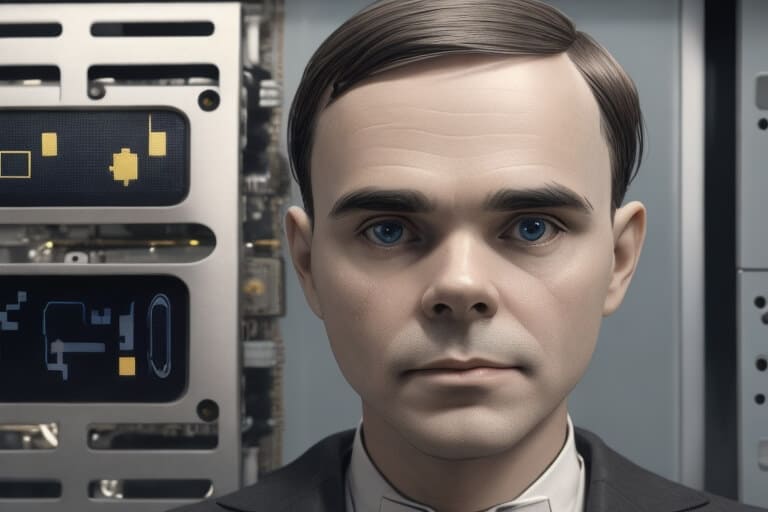
- October 19, 2023
- allix
- Research
In 1950, the brilliant British computer scientist, Alan Turing, introduced an experimental approach aimed at addressing the profound question: can machines possess cognitive capabilities? His proposal posited that if a human conversing with an entity, be it an artificially intelligent machine (AI) or another human, for a mere five minutes, couldn’t distinguish between them, it would signify that AI had achieved human-like intelligence.
During Turing’s lifetime, AI systems were a considerable distance from fulfilling his test’s criteria. He ventured to speculate, stating, “[…] in approximately five decades, it may be viable to program computers […] in such a manner that they emulate the imitation game so convincingly that an average inquirer would not have more than a 70% likelihood of making a correct identification after five minutes of interaction.”
Fast forward over 70 years from Turing’s proposal, and no AI has definitively passed the test as outlined by him. Some systems have approached this milestone, as certain headlines suggest.
A recent experiment examined the capabilities of three extensive language models, among them GPT-4, the AI technology powering ChatGPT. Participants engaged in two-minute conversations with either another human or an AI system, with the AI instructed to introduce minor spelling errors and discontinue the conversation if the tester became overly aggressive.
With these instructions, the AI managed to effectively deceive the testers. When paired with an AI bot, testers could accurately identify whether they were conversing with an AI system only 60% of the time. Given the rapid advancements in natural language processing systems, it is conceivable that AI could surpass Turing’s original test in the next few years.
While the successful completion of the Turing test does offer some evidence of intelligence, it is not a definitive indicator. One issue is the potential for “false negatives.”
Contemporary large language models often explicitly disclose their artificial nature, as ChatGPT does by prefacing answers with “as an AI language model.” Even if AI systems possess the intrinsic ability to pass the Turing test, such programming decisions could override this capability.
The test also carries the risk of “false positives.” As philosopher Ned Block pointed out in a 1981 article, a system could theoretically pass the Turing test merely by being hard-coded to respond in a human-like manner to any conceivable input.
The Turing test focuses specifically on human cognition. If AI cognition varies from human cognition, an expert evaluator may identify areas where AI and humans differ in performance. Turing acknowledged this concern, stating, “This objection is a very strong one, but at least we can say that if, nevertheless, a machine can be constructed to play the imitation game satisfactorily, we need not be troubled by this objection.”
Passing the Turing test offers compelling evidence of intelligence, but failing it does not definitively prove the absence of intelligence. Moreover, the test does not address whether AI systems possess consciousness, the capacity to experience pain and pleasure, or moral significance. According to many cognitive scientists, consciousness encompasses a specific set of mental faculties, including a functioning memory, higher-order thinking, and the ability to perceive the environment and model one’s physical movements within it. The Turing test cannot determine whether AI systems possess these faculties.
There exists no single definitive test for evaluating artificial or human intelligence. At its broadest, intelligence can be seen as the capacity to attain various objectives across diverse environments. More intelligent systems excel at achieving a broader spectrum of goals in a wider range of contexts.
As a result, the optimal approach to monitor the progress of general-purpose AI systems is to gauge their performance across a variety of tasks. Machine learning researchers have devised a range of benchmarks to accomplish this.
For example, GPT-4 demonstrated an 86% accuracy rate in a comprehensive multitask language understanding test, which evaluated its performance on multiple-choice assessments spanning various college-level academic subjects. It performed well in AgentBench, a tool that measures a large language model’s capability to function as an agent, encompassing activities such as web browsing, online shopping, and competitive gaming.
Categories
- AI Education (37)
- AI in Business (62)
- AI Projects (85)
- Research (57)
Other posts
- The Complete List of 28 US AI Startups to Earn Over $100 Million in 2024
- Keras Model
- Scientists Develop AI Solution to Prevent Power Outages
- NBC Introduces AI-Powered Legendary Broadcaster for Olympic Games
- Runway Introduces Video AI Gen-3
- Horovod – Distributed Deep Learning with TensorFlow and PyTorch
- Research Reveals Contradictory Positions Of AI Models On Controversial Issues
- Using AI to Understand Dog Barking
- The Autobiographer App Uses Artificial Intelligence To Record Your Life Story
- Chainer – Dynamic Neural Networks For Efficient Deep Learning
Newsletter
Get regular updates on data science, artificial intelligence, machine