Why Poor Data Destroys Computer Vision Models & How to Fix It
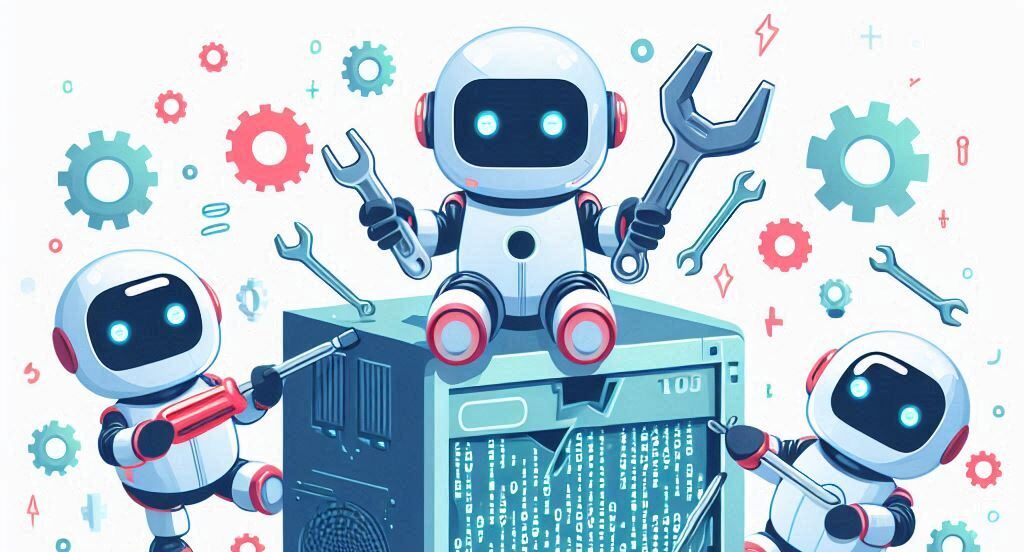
- September 18, 2024
- allix
- AI Education
Artificial Intelligence (AI) is transforming industries, with computer vision (CV) playing a pivotal role in sectors like healthcare, manufacturing, and autonomous driving. However, the effectiveness of CV models relies heavily on the quality of the data they are trained on. Poor data can lead to flawed CV models, resulting in inaccurate object detection, misclassification, and ultimately, a loss of trust and investment. Understanding the impact of poor data on CV and how to mitigate its effects is crucial for harnessing the full potential of AI in visual tasks.
The Impact of Poor Data on Computer Vision Models
CV models learn to interpret and make decisions based on visual data. If this data is incomplete, inconsistent, or erroneous, the model’s predictions can be significantly compromised. Imagine a self-driving car misinterpreting road signs due to poor training data—this could have catastrophic consequences. This highlights the critical importance of high-quality visual data in building accurate CV models.
Bias in CV is another significant concern, often rooted in poor data. If the training data lacks diversity—such as being skewed towards a particular demographic or environmental condition—the CV model may develop biases, leading to unfair or unreliable outcomes. For example, a facial recognition system trained predominantly on lighter-skinned individuals may fail to accurately recognize darker-skinned faces. Addressing bias in CV requires meticulous attention to the data used for training.
The performance of CV models is directly linked to the quality of their training data. Poor data can make a model appear competent in a controlled environment but cause it to fail in real-world applications. This discrepancy can damage the credibility of AI solutions and lead to costly failures.
Handling poor data in CV can be expensive and time-consuming. It often necessitates extensive data cleaning and preprocessing to make the data suitable for model training. Moreover, incorrect insights derived from poor data can lead to flawed business decisions and further financial losses. Investing in high-quality visual data from the outset can save substantial time and resources in the long term.
Common Sources of Poor Data in Computer Vision
Incomplete data is a frequent issue in CV, often arising from human errors, sensor malfunctions, or the merging of incompatible datasets. When critical visual information is missing, CV models cannot fully understand the scene, leading to inaccurate predictions.
Inconsistent data can be another challenge, particularly in CV. This occurs when there are variations in image resolution, color formats, or labeling standards across the dataset. Such inconsistencies can confuse CV models, resulting in unreliable outputs.
Noisy data—data that contains irrelevant or misleading information—can obscure the true patterns in a visual dataset. Noise might stem from sensor inaccuracies, poor lighting conditions, or irrelevant background details. CV models trained on noisy data struggle to identify essential features, reducing their effectiveness.
Biased data in CV often leads to models that perform well on specific demographics or scenarios but poorly in others. For instance, a CV model trained primarily on urban environments might struggle to accurately analyze rural scenes. This kind of bias can lead to skewed results and unfair outcomes in practical applications.
How to Fix Poor Data in Computer Vision
Data annotation in CV involves labeling visual data to provide context and meaning. When done correctly, it ensures that CV models receive accurate and relevant information during training. Utilizing advanced data annotation tools, such as those from Keylabs, can significantly enhance the quality of your visual data.
Data cleaning is the process of identifying and correcting errors and inconsistencies in your CV dataset. This might involve removing duplicate images, filling in missing annotations, and standardizing labeling formats. Clean visual data is essential for developing reliable CV models.
To reduce bias in CV models, it’s vital to actively identify and address potential biases in your visual data. This involves using diverse and representative datasets, applying bias detection algorithms, and continuously monitoring model performance to ensure fairness.
Data augmentation is a technique used to expand your existing CV dataset, making it more diverse and comprehensive. This is particularly useful when dealing with small or biased datasets. Techniques like image rotation, flipping, and synthetic data generation can effectively augment visual data.
Regular data audits are crucial for maintaining high data quality in CV. These audits help catch and correct issues before they impact your model’s performance. Regular reviews ensure that your visual data remains accurate, consistent, and relevant over time.
Using advanced tools for data management and annotation can streamline the preparation of high-quality visual data for CV models. Keylabs offers cutting-edge data annotation tools designed to improve the accuracy and reliability of your AI training data.
Benefits of High-Quality Data in Computer Vision
High-quality visual data enables CV models to make more accurate predictions and decisions, leading to better outcomes in fields like healthcare, manufacturing, and autonomous systems.
When CV models are built on solid data, they gain the trust of users and stakeholders. Reliable outputs encourage greater adoption and investment in AI-driven solutions.
Organizations that prioritize visual data quality can outperform competitors by developing more effective and dependable CV models. Quality data leads to superior business insights and drives innovation.
Investing in high-quality visual data can reduce the need for extensive data cleaning and rework, resulting in cost savings. Additionally, accurate CV models help avoid costly mistakes and enhance operational efficiency.
Best Practices for Ensuring Data Quality in Computer Vision
Maintaining high data quality in CV requires strong data governance policies. These policies should clearly define how visual data is collected, stored, and processed to ensure consistency and accuracy.
Training your team on best practices for data management and annotation is essential for maintaining data quality. Utilizing advanced tools like those from Keylabs can also simplify the data preparation process.
Collaborating with data scientists and domain experts ensures that your visual data is relevant and accurate. These professionals can identify potential issues early in the process.
Continuous monitoring of your visual data is also critical. Regular checks allow you to quickly identify and resolve problems, ensuring that your CV models always have the best data to learn from.
Choose Quality Data Annotation Tools for Computer Vision
Poor data can severely undermine CV models, leading to inaccurate predictions, biased outcomes, and increased costs. By recognizing the impact of poor visual data and taking steps to improve its quality, organizations can fully leverage the power of AI in computer vision.
Investing in high-quality data annotation tools from Keylabs is crucial for building robust CV models. Quality visual data not only enhances model performance but also fosters trust, drives innovation, and gives your organization a competitive edge. Prioritizing data quality is essential for any organization looking to succeed in an AI-driven future.
Categories
- AI Education (38)
- AI in Business (63)
- AI Projects (86)
- Research (58)
- Uncategorized (1)
Other posts
- Meta’s Movie Gen Transforms Photos into Animated Videos
- DIY Projects Made Easy with EasyDIYandCrafts: Your One-Stop Crafting Hub
- Why Poor Data Destroys Computer Vision Models & How to Fix It
- Youtube Develops AI Tools For Music And Face Detection, And Creator Controls For Ai Training
- Research Shows Over-Reliance On AI When Making Life-Or-Death Decisions
- The Complete List of 28 US AI Startups to Earn Over $100 Million in 2024
- Keras Model
- Scientists Develop AI Solution to Prevent Power Outages
- NBC Introduces AI-Powered Legendary Broadcaster for Olympic Games
- Runway Introduces Video AI Gen-3
Newsletter
Get regular updates on data science, artificial intelligence, machine