Exploring DeepMind’s AlphaGo
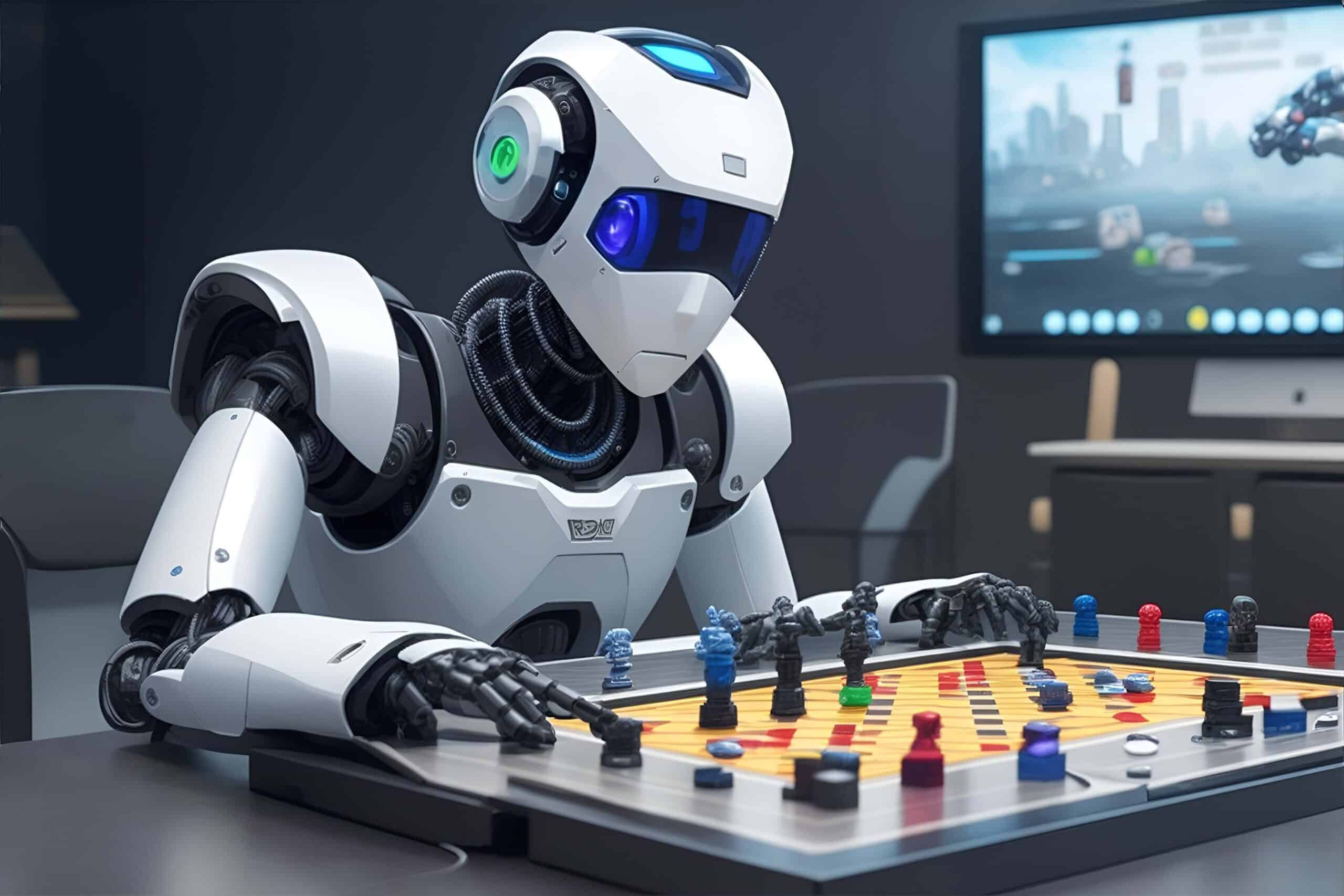
- September 28, 2023
- allix
- AI Projects
Created by the renowned British AI company DeepMind, AlphaGo swiftly rose to prominence in the mid-2010s, showcasing the immense potential of AI in mastering complex tasks that demand intuition, strategy, and an inherent understanding of human cognition.
DeepMind, founded in 2010 by Demis Hassabis, Shane Legg, and Mustafa Suleyman, set out to create AI systems capable of emulating human intelligence. Their ambitious mission was to push the boundaries of AI research and explore the potential of machine learning in conquering complex, real-world challenges.
In 2014, DeepMind achieved an initial breakthrough by developing a neural network capable of learning to play classic video games with superhuman proficiency. This marked the first tangible step on the path to AlphaGo’s creation. While mastering video games was impressive in its own right, the team at DeepMind envisioned something grander – a machine that could conquer not just games but also intricate domains that required deep strategic thinking.
The foundational principles of AlphaGo revolved around the concept of deep learning and reinforcement learning. It was designed to mimic the way humans learn and improve through experience. The base of AlphaGo’s learning process lay in two primary components: the policy network and the value network. The policy network determined the best moves to make in a given position, while the value network assessed the likelihood of victory from a particular board state. AlphaGo’s development was further propelled by a vast dataset of expert-level Go games. This dataset served as a wellspring of knowledge, enabling the AI system to analyze and internalize the strategies and tactics employed by human Go masters.
Conquering the Masters
The most significant event on AlphaGo’s path to fame was its historic showdown against Lee Sedol, one of the world’s top Go players, in March 2016. The five-game match, held in Seoul, South Korea, was more than just a clash of minds; it was a defining moment in the history of artificial intelligence and Go.
The series kicked off with AlphaGo taking an early lead, winning the first three games. Its exceptional ability to evaluate and select moves that befuddled human experts left the Go community, and the world at large, in awe. AlphaGo’s moves seemed not just unconventional but, at times, incomprehensible to those accustomed to human Go strategies. It was a testament to the machine’s deep understanding of the game, forged through extensive training on expert-level games and the application of neural networks.
Lee Sedol, a legendary Go player with numerous titles to his name, managed to claim a victory in the fourth game. AlphaGo clinched the series with a 4-1 victory, securing its place in history. The victory against Lee Sedol was a turning point, not just for AlphaGo but for the entire field of artificial intelligence. It demonstrated that AI could excel in domains requiring intuition, creativity, and strategic acumen, and it opened the door to a new era of AI research and application.
AlphaGo’s Algorithmic
To understand the profound impact of AlphaGo, it’s imperative to delve into the algorithmic that powered its unprecedented success in the ancient game of Go. AlphaGo’s rise to dominance was not merely the result of brute computational force but a harmonious fusion of deep neural networks and a groundbreaking search algorithm known as Monte Carlo Tree Search (MCTS).
AlphaGo was designed to simulate human intuition and expertise in playing Go. The deep neural networks within AlphaGo were the primary tools for achieving this feat. These neural networks were trained on an extensive dataset of expert-level Go games, enabling AlphaGo to recognize patterns, strategies, and subtle nuances in the game that were once thought to be exclusive to human cognition.
The neural networks served two fundamental purposes within AlphaGo: the policy network and the value network. The policy network determined the best moves to make in a given board state, acting as a guide to navigate the intricate landscape of Go. It offered suggestions that often surprised human observers with their novel and creative nature. The value network, on the other hand, assessed the likelihood of victory from a particular board position, helping AlphaGo prioritize moves that maximized its chances of winning.
AlphaGo’s true genius emerged when these neural networks were combined with Monte Carlo Tree Search (MCTS). MCTS allowed AlphaGo to explore the vast decision tree of possible moves and counter-moves in a highly efficient and effective manner. Unlike traditional brute-force methods, MCTS focused on sampling and evaluating promising lines of play, dynamically adjusting its exploration strategy as the game progressed.
This unique blend of machine learning through neural networks and the strategic refinement offered by MCTS gave AlphaGo its uncanny ability to excel at Go. The machine exhibited creativity and strategic depth that surpassed conventional AI systems. It became adept at evaluating complex board positions and discerning not just the immediate consequences of moves but also their long-term implications.
Legacy and Beyond
One notable example of AlphaGo’s influence is the field of self-play reinforcement learning. DeepMind demonstrated that AI systems could learn and improve by competing against themselves, a concept now popularized in various AI domains. AlphaGo also left a profound impact on the world of competitive gaming. It challenged the very essence of what it means to compete against AI and reshaped the relationship between human players and machine intelligence. Games like chess, Dota 2, and StarCraft II have witnessed AI-driven breakthroughs, with developers drawing inspiration from AlphaGo’s success to create advanced AI agents capable of rivaling human players.
Beyond the gaming sphere, AlphaGo’s underlying technologies have found applications in critical real-world challenges. One notable example is AlphaFold, an AI system developed by DeepMind to predict the 3D structures of proteins with remarkable accuracy. AlphaGo’s adaptability and versatility have been demonstrated in climate science, where it aids in climate modeling and prediction. The same algorithms that allowed AlphaGo to analyze complex Go positions have been repurposed to analyze intricate climate data, helping scientists better understand and respond to climate change.
Categories
- AI Education (37)
- AI in Business (62)
- AI Projects (85)
- Research (57)
Other posts
- The Complete List of 28 US AI Startups to Earn Over $100 Million in 2024
- Keras Model
- Scientists Develop AI Solution to Prevent Power Outages
- NBC Introduces AI-Powered Legendary Broadcaster for Olympic Games
- Runway Introduces Video AI Gen-3
- Horovod – Distributed Deep Learning with TensorFlow and PyTorch
- Research Reveals Contradictory Positions Of AI Models On Controversial Issues
- Using AI to Understand Dog Barking
- The Autobiographer App Uses Artificial Intelligence To Record Your Life Story
- Chainer – Dynamic Neural Networks For Efficient Deep Learning
Newsletter
Get regular updates on data science, artificial intelligence, machine