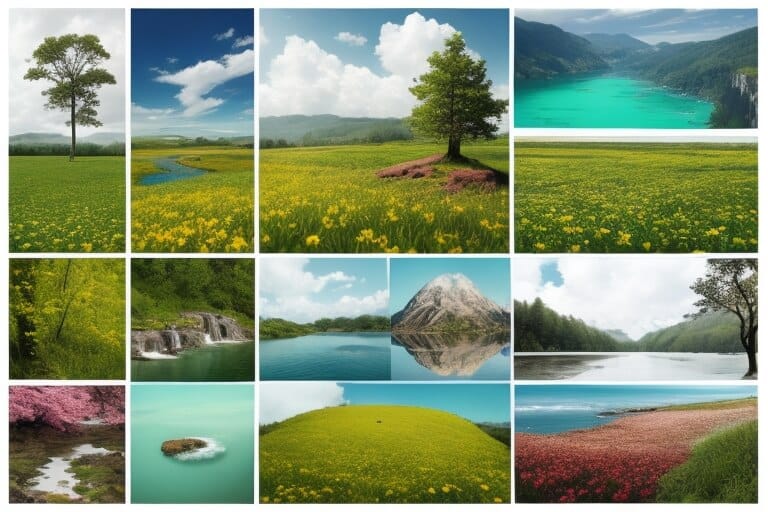
- October 26, 2023
- allix
- Research
With the rise of LensaAI, ChatGPT, and other highly effective generative machine learning models, the internet is experiencing an increasing influx of content, including text, images, logos, and videos, all crafted by artificial intelligence (AI). This content, collectively known as AI-generated content (AIGC), can often be indistinguishable from content created by humans or other computational models.
The widespread adoption of generative AI models has raised significant questions about intellectual property and copyright. Many companies and developers have expressed concerns about the widespread commercial use of content produced by their models. Consequently, they have introduced watermarks to regulate the distribution of AIGC.
Watermarks are distinctive patterns or marks that can be added to images, videos, or logos to clarify their creator and copyright ownership. While watermarks have been in use for many years, their effectiveness in controlling the usage of AIGC remains uncertain.
Researchers from Nanyang Technological University, Chongqing University, and Zhejiang University recently conducted a study to evaluate the efficacy of watermarking as a method to prevent the unauthorized and unattributed dissemination of AIGC. Their research, published on the arXiv pre-print server, outlines two strategies that could potentially enable individuals to remove or counterfeit watermarks on AIGC.
Guanlin Li, one of the paper’s co-authors, explained, “AIGC has become a widely discussed topic in the community. Many companies apply watermarks to AIGC to protect their intellectual property and prevent unlawful usage. One day, we pondered whether we could develop more advanced watermarking for generative models. I suggested, ‘Why not attempt to circumvent existing watermarking schemes? If we can eliminate the watermark, some illicit AIGC might not be recognized as AI-generated. Conversely, if we insert a watermark into real-world content, it could be perceived as AI-generated, leading to significant internet disruption.'”
In their study, Li and his colleagues demonstrated a computational approach to erase or create counterfeit watermarks in AI-generated images. This strategy involves gathering data from a target AI company, application, or content generation service, then employing a publicly available denoising model to refine the data. They used this refined data to train a generative adversarial network (GAN). The researchers found that, after training, this GAN-based model was successful in eliminating or forging watermarks.
Li clarified, “Our study’s concept is relatively simple. To identify watermarked content, the distribution of watermarked content should differ from the original. If we can learn the relationship between these two distributions, we can remove or create a watermark.”
In initial tests, Li and his team found that their approach was highly effective at removing and forging watermarks from various images generated by an AI-based content generation service. Their work underscores the vulnerabilities and the resulting impracticality of relying on watermarking to safeguard the copyrights of AIGC.
Li added, “It’s common knowledge that sophisticated watermarking techniques can be easily erased or counterfeited if the attacker has in-depth understanding of the methods, what’s unexpected is that even with just watermarked content, we can manage to do this. Our method is data-driven, indicating that current watermarking schemes lack security. Frankly, I don’t wish for our work to pose a real-world threat, as it would undermine our ability to manage generative models. I hope it serves as an inspiration for others to devise more robust watermarking methods to defend against such attacks.”
This recent research could potentially motivate companies and developers specializing in generative AI to explore advanced watermarking methods or alternative approaches better suited to preventing the unauthorized distribution of AIGC. Li and his colleagues, inspired by their own findings, are currently working on developing some of these alternative approaches.
Li concluded, “Our current focus is on researching novel watermarking techniques for generative models, not limited to image generation methods but also extending to other model types.”
Categories
- AI Education (37)
- AI in Business (62)
- AI Projects (85)
- Research (57)
Other posts
- The Complete List of 28 US AI Startups to Earn Over $100 Million in 2024
- Keras Model
- Scientists Develop AI Solution to Prevent Power Outages
- NBC Introduces AI-Powered Legendary Broadcaster for Olympic Games
- Runway Introduces Video AI Gen-3
- Horovod – Distributed Deep Learning with TensorFlow and PyTorch
- Research Reveals Contradictory Positions Of AI Models On Controversial Issues
- Using AI to Understand Dog Barking
- The Autobiographer App Uses Artificial Intelligence To Record Your Life Story
- Chainer – Dynamic Neural Networks For Efficient Deep Learning
Newsletter
Get regular updates on data science, artificial intelligence, machine