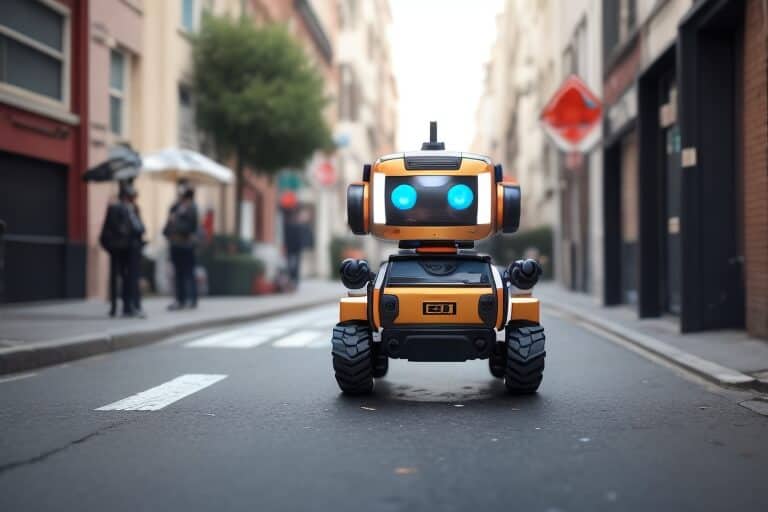
- October 16, 2023
- allix
- Research
Service robots have begun to make appearances in a variety of everyday tasks, such as handling parcel deliveries, providing guidance to the visually impaired, serving the public at airports, and even engaging in construction site inspections, as evidenced in Joensuu. These robots exhibit diverse modes of mobility, utilizing legs, wheels, or even the ability to fly, and possess the capacity to calculate the most efficient or easiest route to their intended destination. For instance, a guide dog robot can be equipped to search for bus schedules and, when necessary, can even arrange for a taxi.
A fundamental challenge persists for these robots: navigating through crowded spaces filled with people. While robots are equipped with cameras and various sensors to observe their surroundings, their movements can often be erratic, featuring abrupt changes in direction and frequent halts. As a result, robots are generally not permitted to operate independently in such congested environments.
The primary issue with contemporary robots lies not in their ability to ascertain destinations or perceive the world around them but in their real-time responsiveness within crowded spaces. Existing methods demand substantial computational resources, making them unsuitable for applications where swift reactions are essential.
In his doctoral dissertation, Chengmin Zhou, MSc, employed reinforcement learning algorithms (RL) to address the challenge of robot navigation, particularly when dealing with multiple moving obstacles. This is especially pertinent in scenarios where a robot needs to navigate amidst a crowd of people and make quick decisions.
The most effective solution identified was a model-free RL algorithm, allowing robots to learn from their past experiences. Post-training, these robots become proficient at handling even challenging situations. The model-free RL algorithm has encountered obstacles, such as slow learning efficiency (convergence). The dissertation focuses on enhancing learning efficiency through two distinct approaches:
- Utilization of data collected during robot operation for training. Real-time data collected during robot operation can be integrated with prior training data, thus bolstering the robot’s training and adaptation.
- Translating environmental information. Sensor data obtained from the robot’s operational environment can be challenging to learn efficiently and accurately. The data requires interpretation or translation to facilitate easy learning for the robot. This knowledge, in the form of a trained model, can then be employed for navigation in similar scenarios.
Robotic navigation has been significantly enhanced from three technical angles: limiting actions (providing robots with a restricted set of choices for their next action), merging real-time and historical data, and harnessing relational data (exploiting the robot’s relationship with obstacles for training). The developed algorithms have undergone testing through computer simulations and within a controlled laboratory environment at Shenzhen Technology University, China.
Chengmin Zhou, MSc’s doctoral dissertation, entitled “Deep Reinforcement Learning for Crowd-Aware Robotic Navigation,” is scheduled for examination on October 19, 2023, at the Faculty of Science, Forestry, and Technology, Joensuu Science Park. The opponent will be Professor Juha Röning from the University of Oulu, and the custos will be Professor Pasi Fränti from the University of Eastern Finland. The public defense will be conducted in English.
Categories
- AI Education (37)
- AI in Business (62)
- AI Projects (85)
- Research (57)
Other posts
- The Complete List of 28 US AI Startups to Earn Over $100 Million in 2024
- Keras Model
- Scientists Develop AI Solution to Prevent Power Outages
- NBC Introduces AI-Powered Legendary Broadcaster for Olympic Games
- Runway Introduces Video AI Gen-3
- Horovod – Distributed Deep Learning with TensorFlow and PyTorch
- Research Reveals Contradictory Positions Of AI Models On Controversial Issues
- Using AI to Understand Dog Barking
- The Autobiographer App Uses Artificial Intelligence To Record Your Life Story
- Chainer – Dynamic Neural Networks For Efficient Deep Learning
Newsletter
Get regular updates on data science, artificial intelligence, machine