Platform Allows AI To Learn From Continuous Detailed Human Feedback Instead Of Relying On Large Data Sets
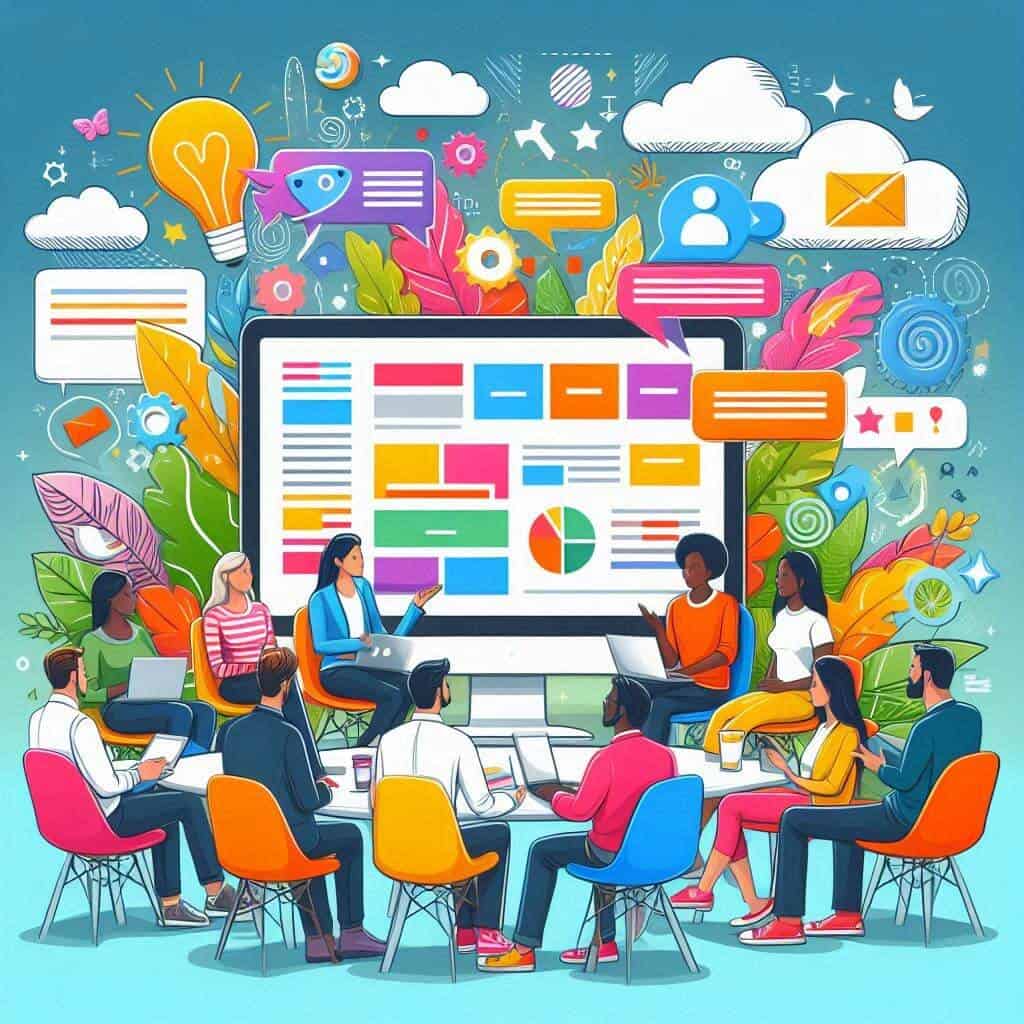
- December 9, 2024
- allix
- AI Projects
During your first driving lesson, you probably had an instructor sitting next to you, giving you instant advice on every turn, stop, and minor adjustment. Over time, these cues and instructions helped develop your experience and intuition, ultimately turning you into a confident and competent driver.
While advances in artificial intelligence (AI) have made self-driving vehicles possible, the training methods used to train these systems are vastly different from those of even the most anxious driving instructors. Instead of relying on detailed, real-time instructions, AI is largely trained using vast datasets and extensive simulations across a variety of programs.
Researchers at Duke University and the Army Research Laboratory have developed a new platform that will help artificial intelligence learn complex tasks similar to human learning. The AI framework, called GUIDE, will be presented at the upcoming conference on Neural Information Processing Systems (NeurIPS 2024) in Vancouver, Canada, scheduled for December 9-15. The research is also available through the preprint server arXiv. “AI still faces challenges when it comes to tasks that require rapid decisions based on limited information,” said Boyuan Chen, a Duke professor with expertise in mechanical engineering, materials science, electrical and computer engineering, and computer science who also directs the Duke General Robotics Laboratory. “Current training methods often rely heavily on large existing datasets, which limits the adaptability of traditional feedback mechanisms,” Chen said. “Our goal was to address this problem by integrating continuous, real-time feedback.”
GUIDE works by allowing humans to monitor the AI’s actions in real-time and provide ongoing feedback. This approach is similar to how an experienced driving instructor provides detailed instructions instead of simply shouting out commands. The initial study of GUIDE involved teaching the AI to play a game of hide-and-seek. The game involved two beetle-like players, one red and one green, both controlled by computers, but only the red player focused on developing his AI capabilities.
The game takes place on a square field with a central C-shaped barrier. Much of the territory remains open only when the red searcher moves into new regions. While the red AI player tracks the other, human trainers offer feedback on its search strategy. Unlike previous attempts that limited input to positive, negative, or neutral responses, GUIDE allows for real-time feedback via a gradient scale controlled by the mouse cursor.
The experiment involved 50 adult participants without prior training, making it one of the largest studies of its kind. The researchers found that just ten minutes of human feedback significantly improved the AI’s performance, achieving a 30% higher success rate compared to current human-guided reinforcement learning methods.
“This compelling evidence underscores the effectiveness of our approach,” said Linyu Zhang, lead author and a first-year Ph.D. student in Chen’s lab. “GUIDE improves adaptability by allowing AI to operate independently in complex, ever-changing environments.”
The study also found that human coaches are mostly needed for short periods. After participants provided feedback, the team developed an AI simulation of the coach based on their ideas. This allows the AI seeker to continue training long after human coaches are no longer available. Training an AI “coach” that is no more skilled than the AI it trains may seem counterintuitive, but Chen notes that it reflects human behavior. “While mastering a task is difficult, assessing improvement is much easier,” Chen said. “Many coaches lead players to victories without winning championships themselves.”
GUIDE also opens up intriguing possibilities by exploring individual differences among trainers. Cognitive assessments administered to all 50 participants revealed that skills such as spatial reasoning and rapid decision-making play a significant role in how effectively someone can guide AI. These findings suggest potential ways to improve these abilities through targeted training, as well as identifying other factors that contribute to successful AI training.
This opens up an exciting opportunity to develop adaptive learning models that focus not only on training AI but also on enhancing human capabilities to build future human-AI collaborations. The researchers aim to create a future where AI learns more efficiently and intuitively, bridging the gap between human intuition and machine learning, and allowing AI to function more independently in information-poor environments. “As AI technologies become more widespread, creating systems that are user-friendly is essential,” Chen said. “GUIDE lays the foundation for more intelligent and responsive AI that can operate autonomously in dynamic and unpredictable situations.”
The team plans to expand their research to include a variety of communication signals, such as speech, facial expressions, and hand gestures, for a richer and more intuitive system for training AI through human interaction. Their work contributes to the lab’s goal of developing advanced intelligent systems that collaborate with humans to solve problems that are beyond the capabilities of AI or humans.
Categories
- AI Education (39)
- AI in Business (65)
- AI Projects (87)
- Research (77)
- Uncategorized (3)
Other posts
- Neck Liposuction Abroad: Say Goodbye to Double Chin, Hello to Defined Confidence
- Dental Veneers Abroad: Get Your Dream Smile for Less
- Endoscopic Brain Surgery Abroad: Safe, Affordable & Advanced Options You Can Trust
- Say Goodbye to Unwanted Moles: Discover Safe, Affordable Mole Removal Abroad
- Mastoidectomy Abroad: Affordable, Safe, and Life-Changing Ear Surgery
- Buccal Fat Extraction: Sculpt Your Dream Look with the Experts at Best Clinic Abroad
- Get Fast Relief from Spinal Fractures: Kyphoplasty Surgery Abroad with BestClinicAbroad
- Curious About Genetic Testing for Fertility? Here’s What You Need to Know (and Why People Trust Best Clinic Abroad to Book It)
- Hip Pain Ruining Your Life? Here’s Why More People Are Getting Hip Replacements Abroad with Best Clinic Abroad
- Breathe Easier: Why Best Clinic Abroad is Your Top Choice for Septoplasty
Newsletter
Get regular updates on data science, artificial intelligence, machine