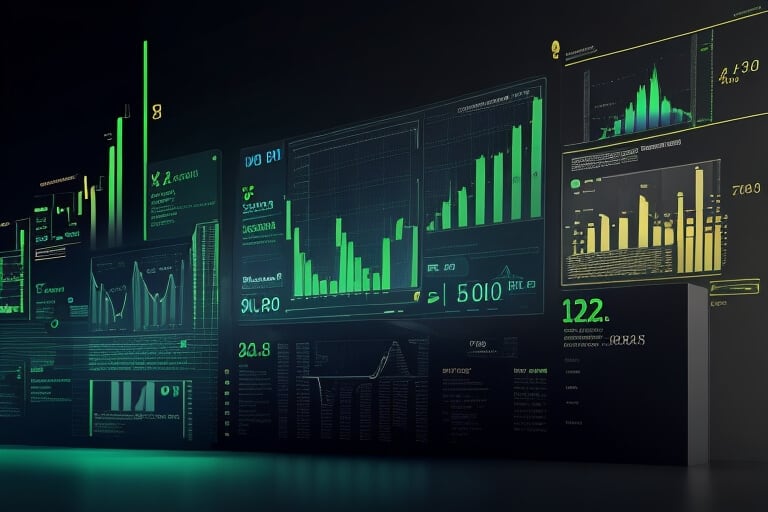
- November 24, 2023
- allix
- Research
Can artificial intelligence tools aid in forecasting changes in stock prices and market volatility? Researchers from South Dakota State University suggest they can. Kaiqun Fu, an assistant professor in SDSU’s Department of Electrical Engineering and Computer Science, and Yangxiao Bai, a graduate research assistant, have crafted an innovative AI-driven model called “ALERTA-Net.” This deep neural network stands out as the first to seamlessly integrate macroeconomic, search engine, and social media data, as detailed in their publication on the arXiv preprint server.
In Fu’s words, “To our knowledge, this marks one of the early efforts to combine social media, macroeconomic data, and search engine information to predict both stock price movements and volatility.”
Given the global significance of U.S. stock exchanges and their role as key indicators of a country’s economic health, accurately predicting their movements remains challenging. Existing methods, such as technical and fundamental analyses, fall short due to inherent flaws.
Technical analysis relies heavily on historical data, potentially overlooking sudden market shifts caused by unexpected events. Fu explains, “Assuming uniformly rational market behavior, this method can inadvertently create an echo chamber, causing trading signals to amplify themselves, eventually becoming disconnected from the actual economic context.”
Fundamental analysis, on the other hand, often neglects the interconnectedness of the broader economy and the stock market, despite taking a more comprehensive approach than technical analysis.
To address these shortcomings, the research team, which included faculty from Virginia Tech and Texas A&M University-Corpus Christi, selected 41 “blue-chip” stocks using the Global Industry Classification Standard. Fu and Bai utilized their expertise in social media information retrieval, employing deep learning—an AI method—to gather and analyze over 7 million tweets discussing these stocks from the social media platform X (formerly Twitter).
Data from Yahoo Finance, tracking the trajectory of the selected stocks over three years, and historical data from the Federal Reserve were also integrated into the model. The results demonstrated the model’s superior accuracy compared to DP-LSTM, a renowned stock movement prediction network, and other baseline models.
Fu and Bai plan to refine their model further by experimenting with new inputs and data sources, considering the integration of audio and video sources. Fu envisions broader applications beyond the stock market, suggesting the model could be used to predict traffic wait times on congested interstates, showcasing the vast potential of this modeling approach.
Categories
- AI Education (37)
- AI in Business (62)
- AI Projects (85)
- Research (57)
Other posts
- The Complete List of 28 US AI Startups to Earn Over $100 Million in 2024
- Keras Model
- Scientists Develop AI Solution to Prevent Power Outages
- NBC Introduces AI-Powered Legendary Broadcaster for Olympic Games
- Runway Introduces Video AI Gen-3
- Horovod – Distributed Deep Learning with TensorFlow and PyTorch
- Research Reveals Contradictory Positions Of AI Models On Controversial Issues
- Using AI to Understand Dog Barking
- The Autobiographer App Uses Artificial Intelligence To Record Your Life Story
- Chainer – Dynamic Neural Networks For Efficient Deep Learning
Newsletter
Get regular updates on data science, artificial intelligence, machine