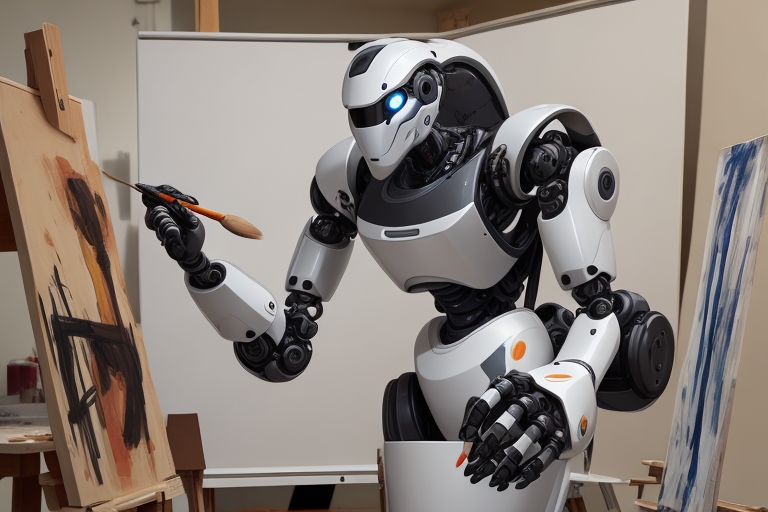
- November 2, 2022
- allix
- Research
Is it a real image or produced by an algorithm? Regularly, the amazing progress of so-called artificial intelligence (AI) blurs a little more the border between reality and its imitation. The first months of 2022 have made it possible to cross an unprecedented stage: having reached maturity, research work is making it possible, in a disturbing way, to transform words into images (photos, drawings, sketches, imitation collages, etc.) on a computer screen. computer.
The most powerful of these technologies are still only in restricted access. But to convince us, others are already available online. Dall-E 2 (a name combining that of Salvador Dalí and the Wall-E robot from the eponymous film) is the best known. It was unveiled in April by the private artificial intelligence research center OpenAI, spearheading these developments. Crayon is a simplified version for the general public. Images from Google, and StableDiffusion, designed by a research group from the Ludwig-Maximilians University of Munich (Germany) with the start-up Stability.AI, are specialized in photorealistic renderings. Those from Midjourney, the American start-up of the same name, have an aesthetic of works of art. In June, the British weekly The Economist even used it to design its front page: a retrofuturistic face on a background of colorful geometric shapes, which illustrated a dossier devoted to “new frontiers of artificial intelligence “.
This current has a name: “text-to-image”. In the first step, the user generates visuals from natural language words and phrases. But the state of research allows us to go much further. By adding terms such as “marker”, “charcoal”, “and watercolor”, but also “Van Gogh” or “Dali”, for example, he can apply the corresponding graphic style to them.
The levels of detail, fidelity to the proposed description, and realism of the textures can be confusing, even for absurd texts. Evidenced by the ability of Imagen to produce the image of a “raccoon wearing an astronaut helmet, looking out the window at night “. A spectacular result, but it requires a lot of trial and error on the text before obtaining a satisfactory result.
Rarely, however, has research work found itself so quickly at the heart of questions of society, art, and the economy. As proof: at the end of August, a table entitled Space Opera Theater, generated by Midjourney and presented as such to the jury, won a digital art contest at the Colorado State Fair (USA). A verdict that immediately aroused the anger of other artists, who themselves had used classic computer graphics software. The human laureate (or rather co-author) had to defend himself by explaining that he had spent 80 hours of work, modifying his text, and correcting elements by hand before arriving at the final work.
Different results depend on the image databases
However, the approach raises questions. The artist depends here on databases on which the algorithms are trained. However, these have implications for their performance, not to mention the biases they may induce. “We can obtain very different renderings between an algorithm trained on a collection of images posted on Facebook and the same algorithm trained on images from Flickr, explains Michel Nerval, co-founder of the digital creation studio U2p050. Some are also much better trained than others. “
The studio released the graphic novel in September Moebia, “drawn” by the VQGan+Clip algorithm from a short story. But we had to test and choose from five databases. “Usually, we would start by entering a sentence written for the book. Sometimes this would give the expected result directly, but sometimes sentences that were too long would ‘lose’ the AI and not work. In this case, we needed rather work by keywords in order to guide the algorithm “, details Michel Nerval.
The “text-to-image” revolution is actually an extension of so-called generative AIs, such as GANs, or antagonistic generative networks, which appeared in 2014. This approach consists in having two algorithms “confront” each other, one creating content, the second judging whether it is acceptable or not. It is also sometimes combined with text input, as in GauGan 2 from the graphics processor giant Nvidia.
The algorithm links a description to an image it has never seen
“The innovation, from the point of view of the text, comes from the Clip model, which makes it possible to represent in a common space the text and the images “, notes Matthieu Labeau, a specialist in automatic language processing at Télécom Paris. Published in January 2021 by OpenAI, Clip is trained on 400 million images and their textual descriptions found on the Internet (captions, metadata), and no longer on images with a summary label (“dog”, “chair”) as in the datasets intended for researchers. The massive aspect of this training material then makes the algorithm able to extrapolate to associate a description with an image he has never seen.
The initial goal of OpenAI was to be able to index and classify images more efficiently. The clip can also be used to search for similar images or to moderate content. But this project led the company to develop the generative algorithm Dall-E, the first version of which was released at the same time as Clip. “Our model is close to that of GPT (natural language processing model also created by OpenAI, editor’s note)consisting of predicting one element at a time (word, article, space, punctuation…, editor’s note) except that instead of being words, these elements consist of snippets of images “, explains the creator of Craiyon Boris Dayma.
For the “image” component, another approach is involved: “diffusion”. This type of deep learning algorithm produces “noise”, i.e. a cloud of random pixels. Then it “denoises” gradually by reorganizing the pixels no longer randomly but taking into account the text describing the desired image. It is the effectiveness of this approach that allows the photorealism of Dall-E 2, poorly managed by the first version (which did not use diffusion) or Imagen.
It is only the beginning. At the beginning of September, a team from the Massachusetts Institute of Technology (Cambridge, USA) presented Composable Diffusion, an improvement in diffusion. “Current ‘text-to-image’ algorithms have some difficulty in generating scenes from complex descriptions, for example when there are several adjectives; elements may be missing from the image “, notes Shuang Li, co-author of the study.
The proposed approach then involves several diffusion models, each taking into account a piece of a sentence. This tends to show, once again, that if the AI demonstrates breathtaking skills, the human remains in control. He is the one who masters the code, publishes it or not, improves it, develops the models, and decides on the training data sets. If there is machine creativity, it (still) depends on humans.
The dark side of technical performance
Impossible, with the Midjourney or Dall-E 2 algorithms, to obtain an image from terms with a sexual or violent connotation. They are set to block them. But StableDiffusion doesn’t have those safeguards… Hence the concerns of Joshua Achiam, a reinforcement learning specialist at OpenAI. In tweets posted on September 10, he welcomes the promises of creativity of “text-to-image”, but fears the influx of violent, shocking, manipulative content.
Another recurring problem in AI: bias. Since these algorithms are trained on content found on the Internet, they perpetuate discrimination of all kinds. Added to this are possible infringements of copyright. The Getty Images photo agency announced at the end of September that it would refuse images created by AI, protected works that could appear in training bases without authorization.
Categories
- AI Education (39)
- AI in Business (65)
- AI Projects (87)
- Research (77)
- Uncategorized (3)
Other posts
- Neck Liposuction Abroad: Say Goodbye to Double Chin, Hello to Defined Confidence
- Dental Veneers Abroad: Get Your Dream Smile for Less
- Endoscopic Brain Surgery Abroad: Safe, Affordable & Advanced Options You Can Trust
- Say Goodbye to Unwanted Moles: Discover Safe, Affordable Mole Removal Abroad
- Mastoidectomy Abroad: Affordable, Safe, and Life-Changing Ear Surgery
- Buccal Fat Extraction: Sculpt Your Dream Look with the Experts at Best Clinic Abroad
- Get Fast Relief from Spinal Fractures: Kyphoplasty Surgery Abroad with BestClinicAbroad
- Curious About Genetic Testing for Fertility? Here’s What You Need to Know (and Why People Trust Best Clinic Abroad to Book It)
- Hip Pain Ruining Your Life? Here’s Why More People Are Getting Hip Replacements Abroad with Best Clinic Abroad
- Breathe Easier: Why Best Clinic Abroad is Your Top Choice for Septoplasty
Newsletter
Get regular updates on data science, artificial intelligence, machine