The Role of Data in AI Learning
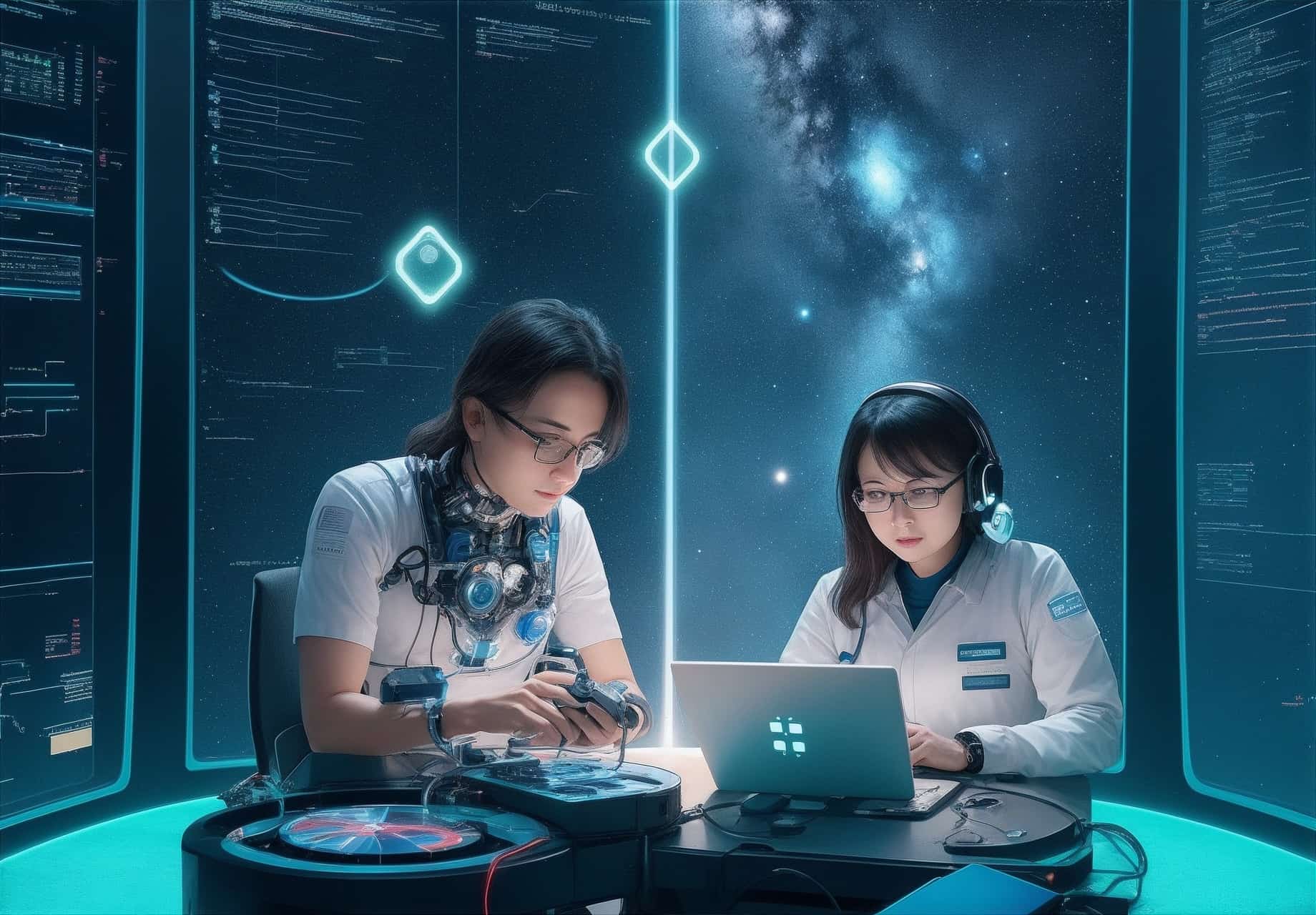
- August 29, 2023
- allix
- AI Education
The fusion of artificial intelligence (AI) and data has catalyzed an era of unprecedented technological transformation. At the heart of this revolution lies an intricate relationship: AI systems depend on data to learn, adapt, and make decisions. As AI applications continue to reshape industries and daily experiences, it’s essential to delve deeper into the pivotal role that data plays in the learning process of AI systems.
The essence of AI’s mission is to replicate human intelligence, which includes the capacity to learn from experiences and make informed decisions. AI accomplishes this through machine learning algorithms, which extract patterns from data and refine their performance iteratively. The fundamental link between data and AI learning emerges here. Just as humans learn from interactions, AI learns from data. The quality, quantity, and diversity of data significantly influence the effectiveness and ethical dimensions of AI applications.
Data as the Cornerstone of AI Learning
In the realm of AI, data functions as the bedrock on which the entire learning process rests. Take, for instance, supervised learning, a prevalent approach in machine learning. In this paradigm, AI models are trained on labeled data, enabling them to make accurate predictions or classifications. The efficacy of the model is intricately tied to the comprehensiveness of the dataset. A dataset with limitations or biases can lead to skewed predictions and suboptimal outcomes.
Data is not solely the foundation; it serves as the fuel propelling AI’s advancement. Continuous exposure to diverse and relevant data ensures that AI systems remain adaptable and responsive to evolving circumstances. This iterative learning process finds manifestation in the development of self-driving cars, where copious amounts of real-world driving data contribute to refining the vehicles’ decision-making capabilities.
Ensuring Reliable and Ethical Data
While the quantity of data is undeniably crucial, the quality of data is equally paramount. Inaccurate, outdated, or biased data can result in erroneous AI outcomes. Imagine an AI-powered healthcare diagnostic system trained on outdated medical literature; its recommendations would be far from dependable. Biases inherent in training data can perpetuate inequalities or discriminatory behaviors in AI applications, ranging from recruitment algorithms to criminal justice systems.
Upholding data quality demands meticulous data curation, validation, and preprocessing. AI developers and data scientists must be meticulous in identifying and rectifying biases and inaccuracies, striving for a comprehensive and representative dataset. Ethical considerations also come to the forefront, as AI systems must be trained on data that adheres to privacy regulations and respects individual rights.
In the pursuit of robust AI learning, the significance of data diversity cannot be overstressed. Diverse datasets mirror the intricacies of real-world scenarios, empowering AI systems to generalize effectively and perform reliably across various contexts. Consider an AI language translation model; if it has solely been trained on formal texts, it will likely struggle to comprehend informal conversations.
Diversity in data equips AI with the capacity to tackle challenges beyond its initial scope. A speech recognition AI exposed to multiple languages will likely adapt more readily when introduced to a new language. This adaptability is pivotal to the flexibility and scalability of AI systems, enabling them to learn continuously and enhance their performance.
Ethics in Data Utilization
As AI’s influence grows, so does the responsibility to wield data ethically and responsibly. The usage of data in AI systems raises profound ethical questions. Should AI make decisions that impact lives? How do we ensure that AI doesn’t perpetuate bias? These questions underscore the intricate interplay between data, AI, and morality.
The responsibility lies with developers, researchers, and policymakers to establish ethical guidelines that govern data usage in AI systems. Transparent decision-making processes and diverse representation in AI development teams are pivotal in avoiding the perpetuation of biases. Frameworks such as “explainable AI” aim to demystify the decision-making processes of AI systems, making them more understandable and accountable.
Another such challenge is data privacy. Striking a balance between the need for data and safeguarding individual privacy is a delicate task. Differential privacy techniques attempt to anonymize data, preserving its utility while minimizing the risk of personal information exposure.
The energy consumption of AI models, particularly large-scale deep learning models, has raised environmental concerns. The computations required to train these models are resource-intensive. Researchers are exploring ways to optimize AI architectures to reduce energy consumption without compromising performance.
Data’s Expansive Reach
The application of data-driven AI learning transcends sectors, leaving an indelible mark across industries. In healthcare, AI systems trained on vast medical datasets aid in diagnosing diseases and predicting patient outcomes. The ability to analyze numerous patient records, genetic information, and medical images empowers healthcare professionals with valuable insights, enabling more personalized and precise treatments.
The realm of finance leverages AI to analyze market trends, identify fraud, and optimize investment portfolios. The colossal amount of financial data is sifted through, unveiling patterns that would be near-impossible for human analysts to detect. AI’s rapid data processing capabilities redefine the speed and accuracy with which financial decisions are made.
AI’s relationship with data extends beyond technical domains into the realm of creativity and art. Creative AI systems are being trained on vast amounts of artistic creations, ranging from paintings and music to literature and architecture. By analyzing patterns and styles across different artistic eras, AI generates new artworks that blend tradition with innovation. However, debates arise regarding the authenticity of AI-generated art and its impact on the creative process.
Categories
- AI Education (38)
- AI in Business (63)
- AI Projects (86)
- Research (58)
- Uncategorized (1)
Other posts
- Meta’s Movie Gen Transforms Photos into Animated Videos
- DIY Projects Made Easy with EasyDIYandCrafts: Your One-Stop Crafting Hub
- Why Poor Data Destroys Computer Vision Models & How to Fix It
- Youtube Develops AI Tools For Music And Face Detection, And Creator Controls For Ai Training
- Research Shows Over-Reliance On AI When Making Life-Or-Death Decisions
- The Complete List of 28 US AI Startups to Earn Over $100 Million in 2024
- Keras Model
- Scientists Develop AI Solution to Prevent Power Outages
- NBC Introduces AI-Powered Legendary Broadcaster for Olympic Games
- Runway Introduces Video AI Gen-3
Newsletter
Get regular updates on data science, artificial intelligence, machine