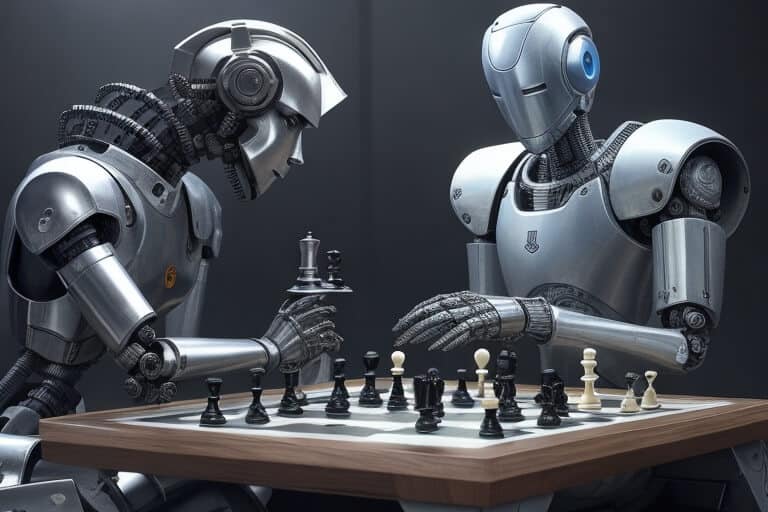
- October 6, 2023
- allix
- Research
A team of researchers hailing from the Singapore University of Technology and Design (SUTD) has achieved a significant breakthrough in the area of artificial intelligence (AI). They’ve harnessed the power of reinforcement learning to conquer the challenge presented by champion Street Fighter players. This innovative research endeavor spawned a sophisticated software designed for intricate movement patterns, utilizing an approach that has previously proven its mettle in board games like Chess and Go. During testing, this novel approach displayed movements that outshone those executed by top-tier human players.
The implications of these findings extend beyond the confines of the gaming world, potentially revolutionizing the domains of robotics and automation. The research article, titled “A Phase-Change Memristive Reinforcement Learning for Rapidly Outperforming Champion Street Fighter Players,” published in Advanced Intelligence Systems, underscores the remarkable strides made by AI in advancing the study of movement.
Desmond Loke, an Associate Professor at SUTD and the principal investigator, emphasized, “Our findings underscore the versatility of reinforcement learning beyond its proficiency in mastering conventional board games. Our program excelled in crafting intricate movements, addressing long-standing challenges in the field of movement science.”
He further elaborated on the transformative potential of this methodology, stating, “If applied judiciously to pertinent research quandaries, this approach holds the promise of expediting progress across a spectrum of scientific disciplines.”
This research marks a watershed moment in the integration of artificial intelligence into the realm of movement science. The potential applications are multifarious, ranging from the development of more autonomous vehicles to the creation of advanced collaborative robots and aerial drones.
Reinforcement learning, a subset of machine learning, entails a computer program learning through experimentation, making decisions, and receiving feedback. For instance, an algorithm can master the game of chess by exploring millions of potential moves, each resulting in either victory or defeat on the board. The program’s purpose is to enable algorithms to learn from their experiences and enhance their decision-making capabilities over time.
In this particular study, the research team provided the computer with a plethora of initial motion data to construct a reinforcement learning program tailored to movement design. The program then iteratively refined each motion, aiming to achieve specific objectives. The computer fine-tuned character movements and strategies until it acquired the expertise to execute moves that surpassed the built-in AI.
Associate Prof. Loke underscored the uniqueness of their approach, remarking, “Our methodology is distinctive in its utilization of reinforcement learning to tackle the challenge of devising movements superior to those executed by top human players. Previously, this was an insurmountable feat with existing methodologies, and it possesses the potential to redefine the scope of movements we can create.”
As part of their research endeavors, the scientists crafted motions that could outwit various built-in AI opponents, confirming the efficacy of their approach.
“Notably, this approach not only proves effective but also demonstrates impressive energy efficiency,” ascertained Associate Prof. Loke. The phase-change memory-based system, for instance, executed motions with a hardware energy consumption of approximately 26 femtojoules (fJ), a staggering 141 times more energy-efficient than existing GPU systems. He further added, “The full extent of its potential for generating ultra-low-hardware-energy movements remains unexplored.”
The focal point of the team’s efforts was to create novel motions capable of swiftly defeating top human players. This necessitated the utilization of decay-based algorithms in motion creation. Algorithmic testing revealed the effectiveness of the AI-generated motions, with several favorable attributes noted as benchmarks of the design system’s success. These attributes included sound game etiquette, adept handling of inaccurate information, the ability to attain specific game states, and the rapid dispatching of opponents.
The program exhibited outstanding physical and cognitive attributes, constituting effective movement design. For instance, the decay-based technique employed in training neural networks required fewer training steps than conventional decay methods, rendering the motions more adept at overcoming opponents.
The researchers envision a future where this methodology empowers them and their peers to engineer movements, skills, and actions hitherto deemed unattainable. Associate Prof. Loke offered a glimpse into this future, stating, “As technology continues to advance, the range of potential applications expands. This includes furthering the capabilities of computers in facilitating high-level competitive tasks, such as in Poker, Starcraft, and Jeopardy, as well as fostering realistic competitions for training professional players, discovering novel tactics, and enhancing the allure of video games.”
Categories
- AI Education (37)
- AI in Business (62)
- AI Projects (85)
- Research (57)
Other posts
- The Complete List of 28 US AI Startups to Earn Over $100 Million in 2024
- Keras Model
- Scientists Develop AI Solution to Prevent Power Outages
- NBC Introduces AI-Powered Legendary Broadcaster for Olympic Games
- Runway Introduces Video AI Gen-3
- Horovod – Distributed Deep Learning with TensorFlow and PyTorch
- Research Reveals Contradictory Positions Of AI Models On Controversial Issues
- Using AI to Understand Dog Barking
- The Autobiographer App Uses Artificial Intelligence To Record Your Life Story
- Chainer – Dynamic Neural Networks For Efficient Deep Learning
Newsletter
Get regular updates on data science, artificial intelligence, machine